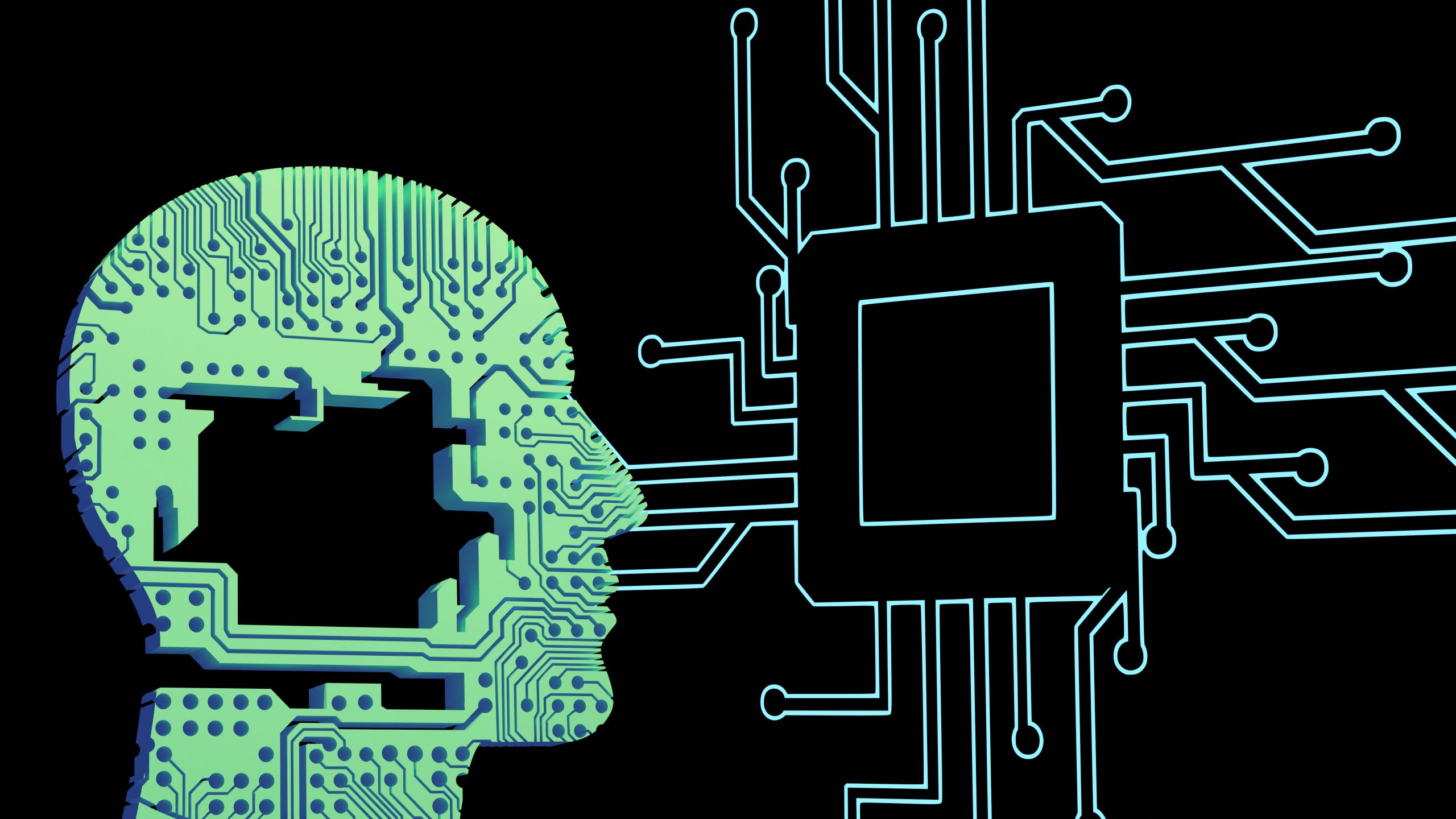
Image sourced by unsplash.com
The rapid integration of AI across our daily digital and social interactions begs for a deeper introspection around AI ethics as a starting point to unravel its harms and benefits. Consequently, there has been considerable business and policy purchase to build safer and more responsible AI technologies, guided by standards and regulatory frameworks for the safe development and deployment of AI. While such discourses have often used the AI supply chain and AI value chain as interchangeable ways of investigation, research suggests they are distinct ontologies. Each offers a separate approach to understanding how AI technologies are currently being made and put to use, which also determines how standards and regulations are framed and implemented.
In the context of Aapti’s broader research on responsible AI, funded by Google.org, our preliminary exploration of the value chain framework has proven useful in understanding how AI development is organised and how responsibilities and accountabilities are distributed among actors. Such a framing offers us an opportunity to think about risk mitigation strategies that are stakeholder and sector-specific. This piece presents a perspective on the value chain framework and shares our early insights into understanding AI development through this lens.
Supply Chain Vs Value Chain
The difference between the supply chain versus value chain approaches is mainly the scope of practices and processes each encompasses. The former primarily alludes to the logistical networks through which AI products are sourced, developed, and delivered, looking at development as a linear downstream flow of resources and processes. Furthermore, the supply chain framework offers a ‘good-dominant’ logic, emphasising the tangible activities that come together in building a product. In contrast, the value chain framework is a ‘service-dominant’ logic that looks at both the tangible and intangible processes. It encompasses inter-relationships across processes, actors, and stages of development, taking a non-linear approach focusing on both the upstream and downstream flow of resources.
The larger scope of the value chain framework, thus can include the broader network of actors, resources, stages, inter-relationships, and situational social, cultural, and economic contexts, providing a comprehensive analytical framework. Such an ontology becomes key in allowing us to scrutinise the co-creative processes and complex relationships inherent in the development and deployment of AI technologies.
Thus, thinking about policy and design-level intervention for risk mitigation within the rubrics of the value chain framework helps account for the modularised nature of AI development. In turn, a value chain approach is instrumental to unpacking the latent process and actors involved in the AI ecosystem, and assign responsibility to create safer and more responsible AI systems.
Why a value chain for AI?
The development of AI systems is a dynamic, multilayered, collaborative process across actors and sources of knowledge. While some developers produce and deploy their AI systems completely in-house, the development of AI is not always so, rather is usually spread across organisations and actors. The nature of the relationship between these actors is also not static but changes depending on the use case, sector of implementation, and how it is being made available to the end consumer. Producing a myriad variety of roles each stakeholder fulfils, depending on their positions across the upstream and downstream spectrum of stages of the AI value chain
General-purpose AI (GPAI) or foundation models which are designed to perform a wide variety of functions have come to dominate AI development. The ability of such models to be trained with general functionalities, and further their ability to learn specific tasks makes AI development using such models not only easier but also more economically viable. However, the usage of GPAI also changes the paradigm of how AI is developed, calling for considered analysis of the value chains of such models.
GPAI model providers (upstream actors) build these models and provide them to companies (downstream actors), who then use the models for their customer-facing applications. However, the resource-intensive nature of developing GPAI models has made the relationship between actors more intertwined and complex. This relationship is further dictated by how such models are made available by upstream actors (as APIs or open-source) which changes the nature of responsibility and accountability each actor has. This makes a linear imagination of assigning responsibility and accountability more obscure, necessitating the need to look beyond just the development of the AI system and take into account release strategies and inter-relationships. Further, looking at the development of AI suggests that the modularised process of making such systems cannot be simply comprehended by looking at it as a linear flow of goods and services.
A value chain ontology thus helps us understand how AI development is organised and how responsibility is distributed among different actors. Further, it lends significance to the social locations and positionalities of developers, as well as allowing us to look at inter-relationships across the actors and stages without restricting us to a linear imagination. This approach then enables policy regulations, responsible AI frameworks, and mitigation strategies informed by normative approaches, be operationalised at different stages of the AI value chain.
Mapping the AI Value Chain
By adopting a value chain framework, we can divide the development of AI systems into various phases and take into account important players participating at each stage and other relevant factors impacting each stage. In landscaping the AI value chain we have divided it into three main stages, each containing distinct activities carried out in the development of an AI system,
- Pre-development involves the modelling of algorithms and building a dataset
- Development involves putting the model to use by testing, training, and validating it
- Adaptation involves integrating the model into user-facing software or services and continuously learning from user interactions to improve its functionality.

The AI Value Chain
Source: Aapti Analysis
The value chain might change with different business models, typologies of AI models, or varying sectoral implementation and use cases. However, this broadly classified value chain framework with clearly defined stages and actors, can be dynamic and modular enough to encompass different business typologies, AI models, and sectors. However, the limitation of this value chain is that it doesn’t represent the computing layer of AI – a critical hardware component riddled with inequities among which bias does not feature. Aapti’s research peels away at the model design questions as a lever to investigate bias within the AI value chain.
Our research at Aapti has been able to disaggregate and understand the different processes and roles stakeholders perform across the value chain. With this understanding, we hope to further be able to pinpoint sources of bias within the AI value chain and design strategies informed by stakeholder- and stage-specific risk mitigation considerations. Our research shall further explore the variances in the AI value chain for different sectors, like finance, healthcare, and education, understanding such variances should also help in adopting a sectoral lens to our recommendations.