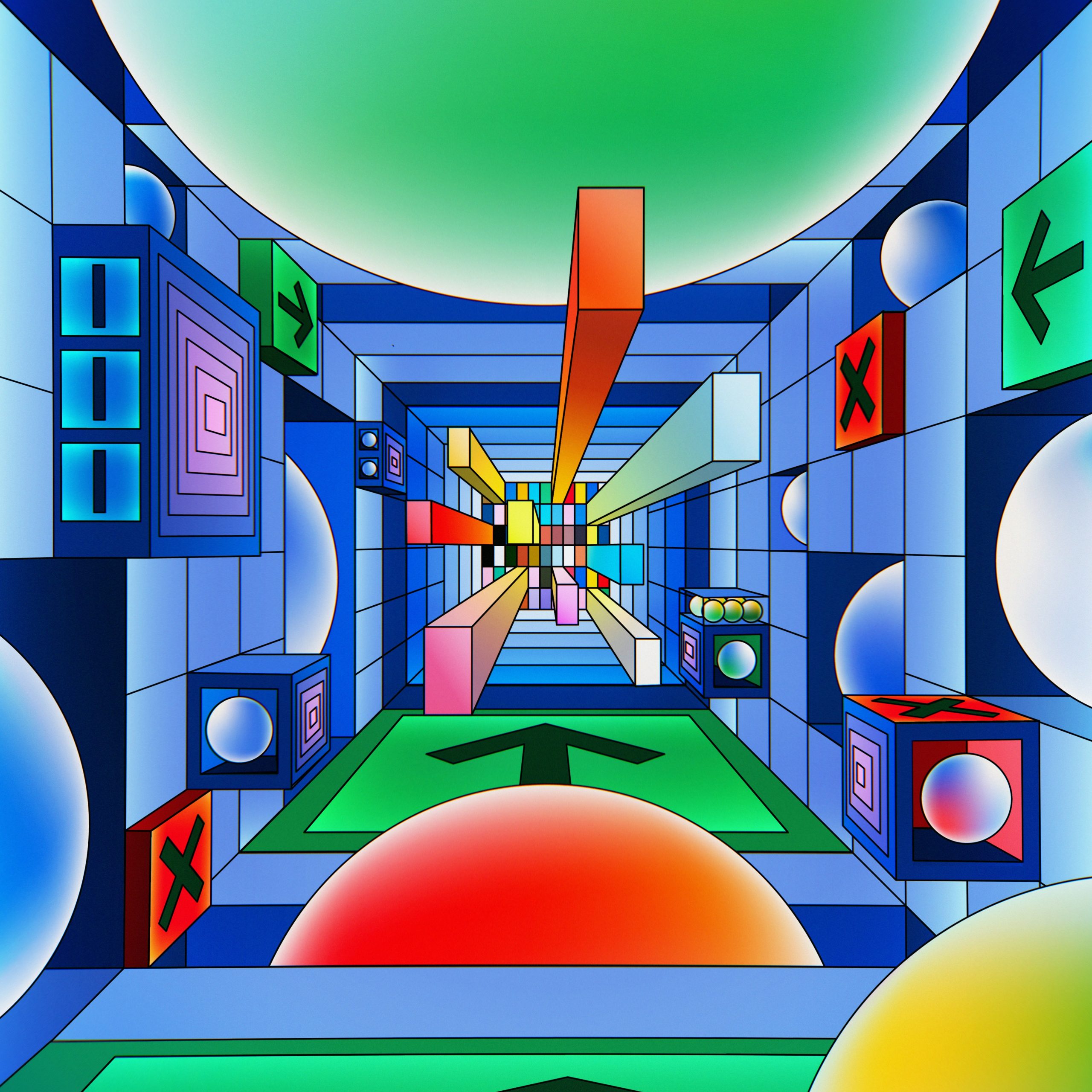
Image sourced by unsplash.com
The framework principally aims at capturing pain points within the AI value chain where bias is entrenched. The framework is based on a value chain ontology, looking at the development and deployment of AI systems, mapping processes and actors, which play both active and passive roles in building such systems. Grasping these complex and multilayered flows of resources and responsibilities is crucial to gaining insight into how AI systems are currently being built, allowing us to not only pinpoint sources of bias but also think about focused mitigation strategies.
The framework additionally adopts a sectoral lens by looking at how the AI value chain differs and the manifestations of various categories of bias across finance, health and education. These sectoral offshoots draw from the general framework and help us understand sector-specific AI value chains and biases, allowing us to adopt sectoral attention to our mitigation recommendation.
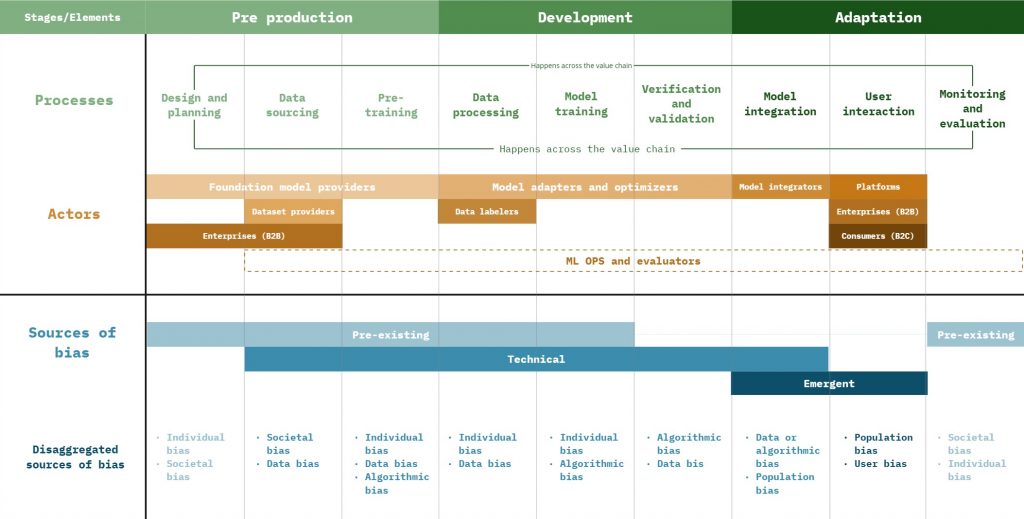
About the Framework
What does the framework represent?
- The framework aims to demonstrate where biases get entrenched at different stages of the AI value chain.
What is the framework mapping?
- The value chain maps the various processes and actors that are part of building AI systems across three stages: pre-development, development, and adaptation
- The framework divides biases according to their various sources into three broad categories: pre-existing, technical, and emergent, further mapping where in the value chain these biases get encoded into AI systems
- The framework also maps the differences in the value within three different sectors (finance, health, and education), and the manifestations of bias categorised according to origin within specific value chains.
Who is the framework for?
- The framework is for tech developers, civil societies, researchers, academicians, and policymakers to help them navigate the complexity of value chains and a specific type of risk under consideration, such as bias and its sources
- The framework aims to comprehensively break down AI systems into its development and deployment stages, highlighting various biases, their sources, and their manifestations.
- Going forward, we shall supplement this framework with mitigation strategies, in accordance with the identified sources and manifestation of biases.
How did we build the framework?
- The framework adopts a value chain ontology to unpack the development and deployment of AI. It includes the broader network of actors, resources, stages, their inter-relationships, and their situational social, cultural, and economic contexts, allowing us to scrutinise the co-creative processes and complex relationships inherent to AI technologies
- The framework borrows from literature to categorise bias within AI systems according to the origin or sources underpinning bias. Identify how and when different sources of bias impact the system within the AI pipeline
- Our framework combines the different categories of bias and the AI value chain to map processes within the value chain where biases may get entrenched, alongside mapping the various sources of bias and actors who are responsible for those processes
- We further disaggregate the larger framework drawing out variance in the development of AI systems within sectors of interest, such as finance, health, and education. The differences lie in the processes followed and how various actors are involved at different stages of the value chain.
- Lastly, through the lens of manifestations of bias within AI systems, we map them according to their probable sources and locate the stage of the value chain at which they get encoded within AI systems
How to read the framework?
The framework is divided into two main parts,
- The general frameworks, a holistic overview that demonstrates the general purpose AI value chain (processes and actors) and the sources of bias within it
- The sector-specific frameworks illustrate how domain-relevant value chains evolve across finance, healthcare, and education, highlighting respective nuances and departures from the GPAI value chain
The general framework
The general framework is divided into two sections.
The first section of the framework contains rows and columns that signify the following:
- The columns represent the different stages of the value chain;
- Pre-development: This stage extends from the modelling of algorithms and building a dataset
- Development: This stage involves putting the model to use by testing, training, and validating it
- Adaptation: This stage involves integrating the model into user-facing software or services and continuously learning from user interactions to improve its functionality.
- The rows represent the different elements of significance such as:
- Process: Identifies the various steps and actions involved at each stage of the value chain.
- Actor: Highlights the stakeholders responsible for carrying out specific processes within the value chain.
- Sources of bias: Maps where the different categories of bias get entrenched across the stages of the AI value chain.
The second section of the general framework briefly maps the variances in the processes and actors of the value chain depending on the sector for which the AI system is being developed.
The sector-specific frameworks
- We focus on three sectors – finance, health, and education.
- Each sector is mapped on a different table, where the columns are the various stages of the value chain, and the rows represent different elements
- Process: Maps how the steps and actions vary for different sectors in the development of an AI system,
- Actors: Maps how different stakeholders are embedded at different stages within the different sector-specific value chains
- Bias manifestations: Maps different examples of bias according to what stage they are likely to get embedded in an AI system.
Please note: The framework is a work in progress and will be updated periodically.
Resources:
- Attard-Frost, Blair, and David Gray Widder. “The Ethics of AI Value Chains.” arXiv.org, July 31, 2023.
- Chmielinski, Madhulika Srikumar Jiyoo Chang, Kasia. “Risk Mitigation Strategies for the Open Foundation Model Value Chain – Partnership on AI.” Partnership on AI, July 19, 2024.
- Simon Caton and Christian Haas, “Fairness in Machine Learning: A Survey,” arXiv.org, October 4, 2020
- Ferrara, Emilio. “Fairness And Bias in Artificial Intelligence: A Brief Survey of Sources, Impacts, And Mitigation Strategies.” arXiv (Cornell University), January 1, 2023.