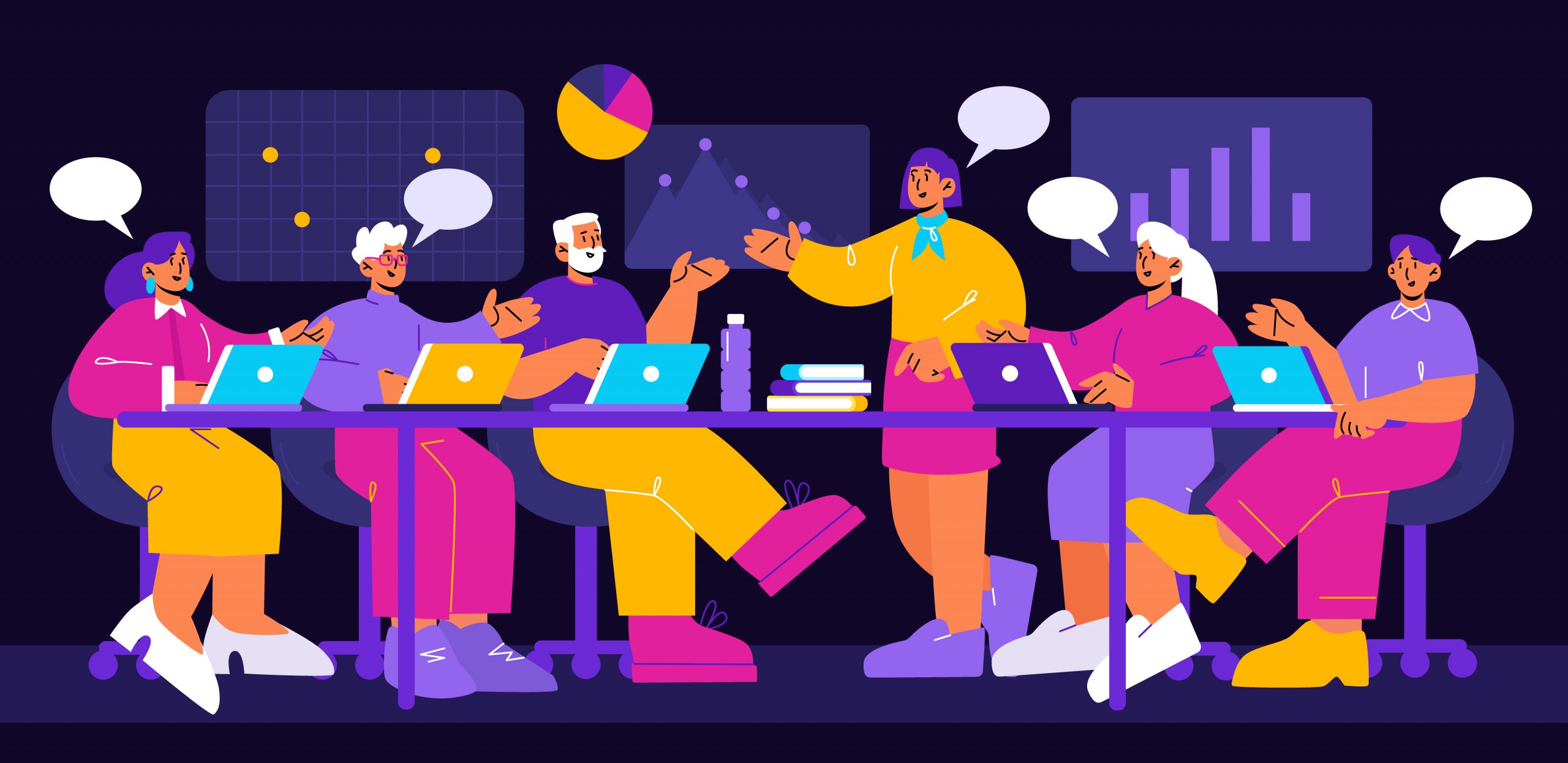
Image sourced by www.freepik.com
Communities of practice (CoP), as explored by Etienne Wenger and Jean Lave in the early 1990s, evolve from the perspective that the acquisition of knowledge has a communal facet, where the knowledge produced within a group is a shared resource and the process of acquiring the knowledge is social. According to Wenger, CoPs are characterised by three key elements: a ‘domain’ of knowledge or activity that creates a common identity, a ‘community’ of members who care about the domain, and a shared ‘practice’ that members develop to be effective in the domain. These elements distinguish CoP from other types of communities, emphasising the importance of a shared focus, mutual engagement, and collaborative learning.
As public problems become increasingly complex, there is a realisation that the traditional structure of learning and knowledge creation is slow and hierarchical. Further a stronger push for public participation and transparency in decision-making have been instrumental in reimagining current structures for policy and development action. Within these contexts, CoP as a method of learning and knowledge creation have gained momentum as, arguably, better levers for the collection, use and dissemination of data and information.
By synthesising and stewarding a community of practice around a specific public issue, CoP can facilitate cross-institutional and cross-sectoral collaboration that is often lacking in traditional knowledge structures. Such communities become an important instrument for bringing together a wide group of stakeholders with different knowledge perspectives. Examining the problem from various perspectives not only fosters a deeper understanding of the problem and provides for multi-dimensional solutions but also plays a crucial role in building trust and promoting collaborative problem-solving across different agencies, institutions, and organisations.
Navigating the Duality of AI
While the adoption and use of AI technologies are ubiquitous across sectors, it is not without its contentions. To begin with, it is important to recognise that the AI wave is backed by technocratic utopian narratives that evolve rapidly, leaving limited room for governance interventions. A key issue that stymies the potential to realise visions of responsibility and fairness within AI systems is the definitive lack of transparency in how such systems are developed and deployed.
The emergence of community-centred AI technologies has proved to be effective in building safer online environments. Organisations like Tattle generate value through AI tools and datasets to tackle misinformation and harmful content. Community-driven projects like Openmined have also been able to build tools which allow for sharing data and machine learning models, without compromising privacy. Open, free data repositories such as Common Crawl have also democratised access to training datasets, allowing for more transparent development of AI models. Such endeavours demonstrate the emancipatory capabilities endowed in AI but require concerted effort and direction to translate similar visions at scale. Consequently, a community-led approach to answering the question of ‘what is responsible AI’ is also necessary. Such an approach emphasises values such as shared ownership and democratic governance of AI systems which are best explored within the aegis of a CoP model of inquiry.
Values of the CoP approach
The lacuna posed by a lack of dedicated research looking at both the risks and opportunities afforded by AI, as well as its implications for online safety, provides an opportunity to co-learn and co-create expertise around the question. In this regard, the CoP approach is critical to realising the values that define discourses around responsible AI. Such values are best embodied through the following CoP levers:
- Collective expertise – The aim of building a CoP is twofold. One is to bring together individuals directly engaged in the domain of responsible AI and building AI technologies. Two is to also build a community that espouses transdisciplinary knowledge and perspectives which are necessary in dealing with the question of AI and safety.
- Cross-sectoral and cross-institutional collaboration – A key value that is driven by a CoP is its ability to connect people across sectors and institutions. Such a collaborative environment is able to accommodate stakeholders across the value chain, connecting funders, organisations, and communities to collectively think about AI governance.
- Building trust and cooperation across stakeholders – Collaborations across varied networks of AI stakeholders are critical to building trust, and consensus and identifying shared responsibilities.
The informal knowledge structure of a Community of Practice (CoP) provides a dynamic ecosystem that fosters a shared understanding and a common vocabulary. This collective intelligence enables critical examination of AI’s potential harms and opportunities, particularly in the realms of security and digital integrity. By uniting diverse perspectives and expertise, CoPs cultivate an environment of trust and collaborative problem-solving, paving the way for innovative and responsible AI applications. Ultimately, this approach not only enhances our ability to address complex public challenges but also builds safer and more equitable digital experiences across sectors, embodying the true spirit of democratised knowledge and collective progress.