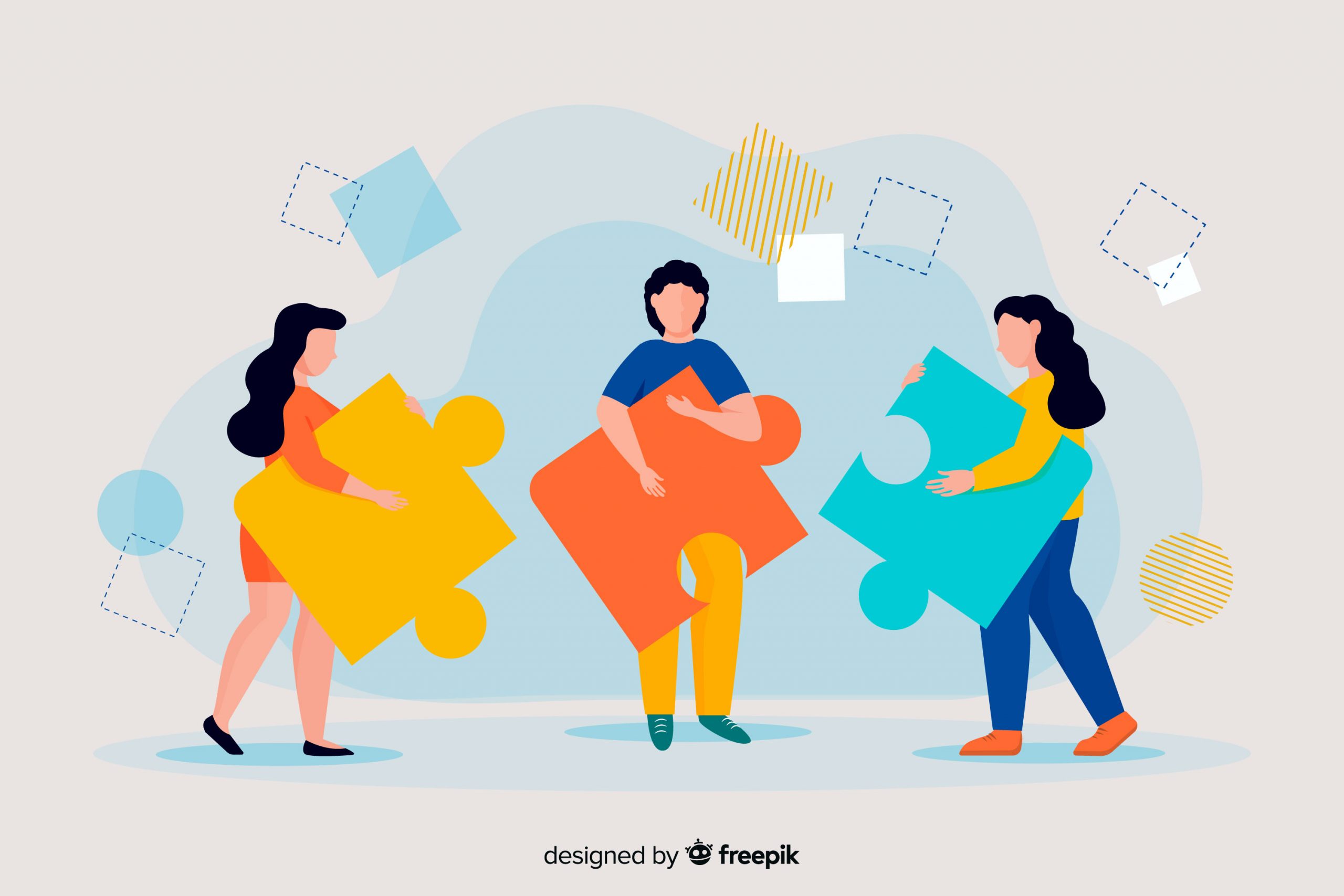
Data is a relational good, meaning that although data might be generated by or pertaining to an individual, its use and insights derived from data, both positive and negative, have an impact on the collective as well. Data must therefore be governed in a manner which balances individual as well as group interests.
Aapti Institute has been studying different models of data stewardship to explore how collective data governance can be facilitated effectively in different situations. Of the several models of stewardship that we’ve explored we found, through our research, that the data cooperative model shows great potential for the collective governance of data.
Data cooperatives are technical and institutional layers which mediate data flows between those who generate data (members) and those who use it (members, the cooperative itself and external parties). Put simply, similar to how traditional cooperatives pool resources or labour to achieve their members’ goals, data cooperatives pool data for specific purposes defined by their members. They embody the principles of the cooperative structure, which are:
1. Voluntary and Open Membership
2. Democratic Member Control
3. Member Economic Participation
4. Autonomy and Independence
5. Education, Training, and Information
6. Cooperation among Cooperatives
7. Concern for Community
With an eye to exploring whether data cooperatives can help empower women and address power asymmetries, Aapti and Data2X partnered to explore how the traditional organisational structure of a cooperative can be used by women to make effective decisions around their data. Discussions in Room 9 of the Brookings Institution’s 17 Rooms initiative helped us take our work forward by contextualising gender data cooperatives, defining their foundational elements and identifying the pathways to operationalise them.
During our inquiry, however, we found that there were very few real-world examples of data cooperatives, especially in the Global South. Furthermore, there existed no data cooperatives that were solely dedicated to serving women, whose experiences and labour tend to be invisibilised due to lack of data.
In order to address this gap, we focussed our efforts towards facilitating the establishment of women’s data cooperatives, especially in the Global South. This phase of our journey started with our work with Megha Mandli, a cooperative falling under the aegis of SEWA, composed of women farmers in Surat, Gujarat. We initiated the process of building a data layer around their existing organisational structure in order for these women to obtain access to credit.
Equipped with learnings on the practical challenges of establishing a data layer in an existing cooperative and other insights from the field, we launched an incubator for women’s data cooperatives. Data Co-ops in Action was thus conceived as a support network for setting up and supporting women’s data cooperatives across the globe. Our first cohort is supported by the Uncommon Collaborations Grant, an initiative supported by The Rockefeller Foundation and Dalberg Catalyst.
As part of the Uncommon Collaborations programme, we deployed a global search strategy to identify women’s organisations that were looking to establish a data layer. Following a rigorous selection process, we selected two organisations, one based out of Tanzania and the other operating in Papua New Guinea.
Our grantees have received microgrants between $5,000 and $10,000 to kickstart their journey of establishing their data layers. To help them navigate this process, we have been carrying out virtual co-design workshops to collaboratively brainstorm with our grantees to outline the technical and governance structures for the data layer. In January 2024, we intend to finalise the structure of the data layer during in-person co-design workshops.
This will then translate into roadmaps which the organisations will follow in operationalising their data layers. The handbook on building a data cooperative, which we are co-authoring with Open Data Manchester will also serve as an aid in the operationalisation of the data layer.
Although this journey has been largely positive, we have encountered (and continue to encounter) several practical challenges that have emerged as important learnings for us. We have documented these learnings as a three-part series. In the next two parts, we will delve into how we designed and deployed our search strategy and the key learnings from this first cohort.