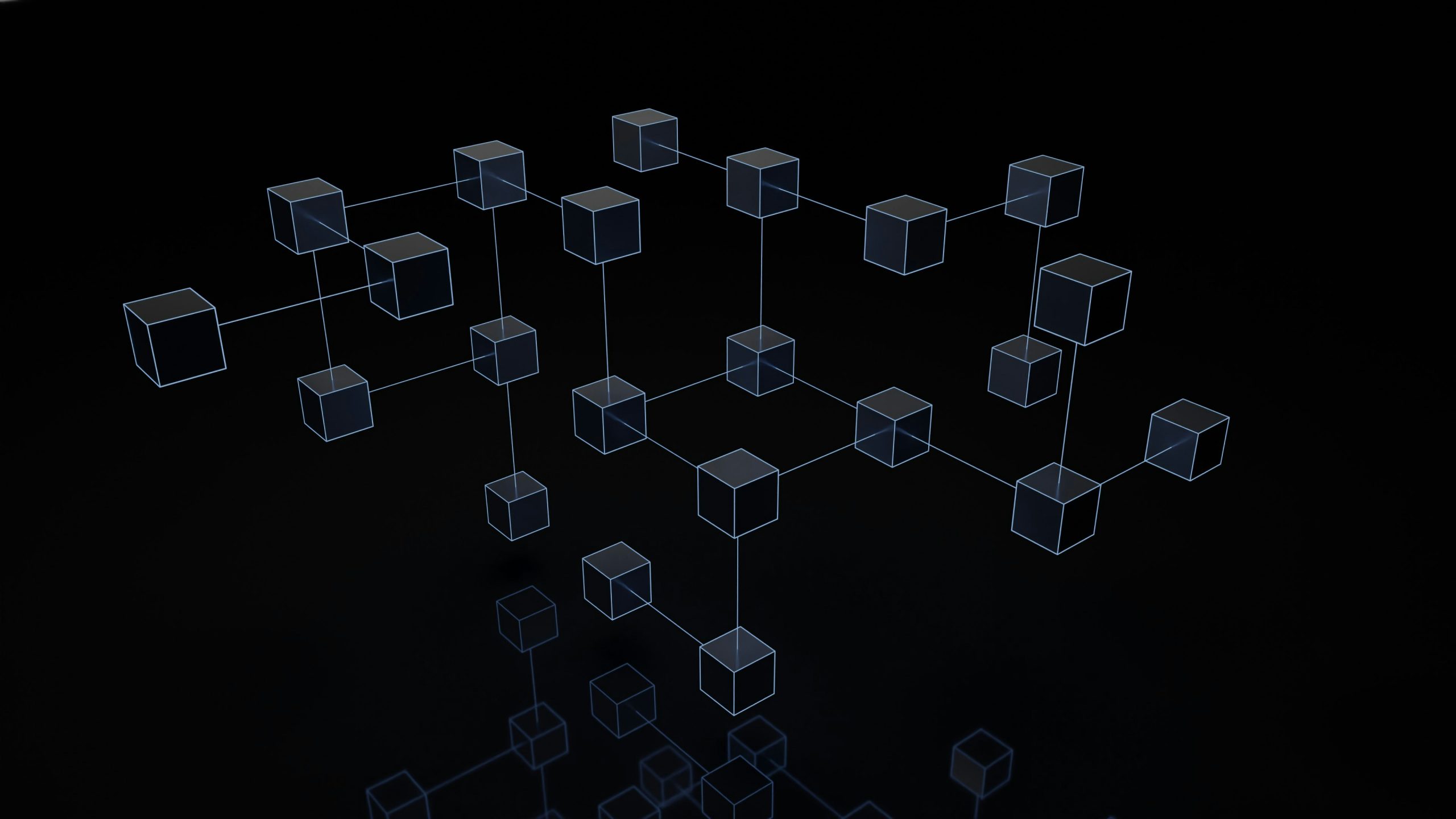
Image sourced by unsplash.com
Responsible Robots: Beyond Metal and Morals
Responsible AI (R-AI) emphasises trustworthiness, ethical design, and risk mitigation to protect both people and the planet. Although we have seen the word “ethics” commonly used in conjunction with AI systems, it is acknowledged that not everyone shares the same ethical standards. Instead of defining ethics for others, being responsible means understanding the impact of actions and safeguarding the rights and choices of individuals or groups. Organisations must establish transparent AI ethics principles and ensure that their AI systems are both responsible and trustworthy, as these concepts are interdependent.
The evolution of responsible AI traces back to early concepts like the Turing Test and Asimov’s Laws of Robotics. By the 2000s, responsible AI expanded to include fairness, transparency, accountability, and societal impact. Today, regulatory frameworks like the EU AI Act and the U.S. NIST framework focus on making AI systems more human-centric and trustworthy. However, no universal definition or framework for responsible AI exists. Against this backdrop, Aapti’s research aims to delineate R-AI practices and principles that are best suited to respond to the twin priorities of innovation and safety that are at the core of India’s AI discourse.
Wrestling with AI: The Challenge of Responsible Design
Key technical principles in responsible AI include fairness (avoiding bias), transparency (clear processes), accountability (assigning responsibility), privacy (protecting data), and robustness (ensuring reliability). These principles collectively aim to build AI systems that are both ethical and technically sound. However, many responsible AI frameworks lack practical guidance, making implementation difficult, especially for those without expertise. Additionally, issues like divided accountability, disciplinary silos, and the gap between technical and non-technical teams complicate efforts to create well-rounded AI systems. To bridge this gap, responsible AI frameworks must be broad, adaptable, and participatory, incorporating input from a range of stakeholders. Impact assessments, such as the IEEE 7010 standard and UNESCO’s Ethical Impact Assessment Toolkit, offer a promising method to evaluate AI’s effect on human well-being and its intended use cases and can help operationalise responsible AI practices.
Even AI models built with responsible frameworks face significant challenges such as biased outcomes, opacity, and unintended consequences. For example, a 2018 MIT Media Lab study revealed that facial recognition systems had error rates of up to 34% for dark-skinned women, while errors for light-skinned men were below 1%, highlighting bias in training data. Another challenge is the opacity of AI systems, particularly in healthcare, where algorithms that analyse medical images to diagnose diseases like cancer often provide decisions without clear explanations, leaving doctors and patients uncertain about the reasoning behind diagnoses. Lastly, unintended consequences, such as social media algorithms amplifying harmful content, demonstrate how AI systems designed to boost engagement can inadvertently cause societal harm. These challenges illustrate the complexity of ensuring AI operates responsibly across all applications. These limitations highlight the need for continuous improvement in responsible AI design and governance. By focusing on flexibility, accountability, and collaboration, responsible AI frameworks can better address the ethical, social, and technical challenges of AI systems while balancing the evolving risks AI poses.
In that regard, revisiting how we understand and frame responsible AI (R-AI) is necessary and we must begin by looking at what have been some of the hurdles in terms of the implementation of R-AI thinking into AI development. Only by comprehending the current predicaments in the R-AI ecosystem, we can try to reimagine how to calibrate future R-AI research and implementation. Additionally, by adopting a techno-societal framing for our research, we aim to address the problems of flexibility, accountability and collaboration. Such framing is rooted in the idea that design-specific and participatory approaches to R-AI are key to good AI governance.
The Achilles’ Heel of Responsible A: Where It Falls Short
Research looking at the scholarly exploration of responsible AI (R-AI) shows that it remains fragmented, while the organisational adoption of R-AI frameworks remains inconsistent allowing gaps to exist. A primary problem which can be identified is the translation of normative principles into design-level language. The current esoteric, complex and multi-nodal process of AI development, means sweeping frameworks get lost across the myriad of actors and processes that participate in building AI systems, and thus have limited impact on the development of AI technologies.
While most R-AI frameworks put blanket responsibility for the ethical behaviour of AI systems on their designers and developers, research on role-relevant strategies for actors has been nascent, often focusing instead on abstract stakeholders. What has complicated this approach is the modularization of AI development and the involvement of multiple actors in building AI systems creating additional challenges for establishing clear governance mechanisms and producing regulatory gaps. Making it difficult to effectively assign responsibility and accountability for adhering to such frameworks among the various stakeholders involved.
This invites us to rethink how we wish to regulate and inform AI design to address the risks that creep in while enhancing its inherent benefits. At Aapti, our research on Responsible AI, particularly in mitigating biases in AI systems, has led us to explore how AI frameworks, principles, and regulations can be reimagined. We believe that revising current and future R-AI frameworks to align with the AI lifecycle in mind can enhance the ecosystem by shifting the focus from informing products or technologies to informing the processes and stakeholders involved in AI development. An approach which is grounded in a deep understanding of the current state of AI development and its complexities. Such an approach allows us to look at multiple actors and build workable collaborative strategies to address risks at their source.
Our Responsible AI Recipe: Crafting The Perfect Blend
The value chain ontology in this regard has proven to be of significant contribution towards framing R-AI strategies that go beyond normative considerations to surface role-relevant, stage-adaptive models for AI governance. The ontology allows us to disaggregate the complex multi-nodal nature of AI development into stages and further into processes and steps, encompassing active and passive stakeholders and demystifying the roles each performs. Mapping sources of risks within the stages of the value chain then allows us to develop mitigation strategies which are configured to how actors and processes interact at each stage. What’s more, such an ontology in helping us understand how different actors play different roles in development permits us to concretely allocate accountability and responsibility among stakeholders relative to their interaction within the AI value chain.
Reframing Responsible AI within the AI value chain can help address many of the challenges it has encountered. By embedding responsible practices throughout the lifecycle of AI development, we can chart a course toward creating AI technologies that are more fair, transparent, accountable, safe, and robust—aligning with the goals envisioned in Responsible AI discourse.
From the AI Wild West to Responsible AI: Our Strategic Blueprint
To truly realise this vision, Responsible AI frameworks must be expansive, addressing AI’s impacts across ethical, social, and economic domains, while integrating specific tools such as bias mitigation, human-in-the-loop systems, user consent management, data minimisation and privacy-by-design among others. These frameworks should not remain abstract but must be operationalized, with principles that translate into actionable strategies throughout the AI lifecycle, ensuring accountability at all governance levels. Flexibility is crucial, allowing adaptation to diverse AI systems, use cases, and organisational contexts, while fostering shared understanding and collaboration.
An iterative approach is key, applying the framework continuously as AI systems and societal contexts evolve. Clear guidance and documentation are essential, enabling even moderately skilled users to apply, customise, and troubleshoot the framework effectively. Participation from a broad range of stakeholders, including those directly impacted by AI systems, is critical to encourage interdisciplinary collaboration. This approach ensures that Responsible AI balances technical precision with a holistic understanding of AI’s far-reaching impacts on human well-being, moving beyond a narrow focus on isolated issues like bias and privacy.