For citizen science projects to be rooted in encouraging bottomup forms of participation and empowering communities, we need to make sure that their involvement is made technologically feasible. Implicit biases in design decisions limits an individual’s or group’s ability to access or use technology reinforcing existing gaps of peoples’ environmental advocacy abilities and exacerbating environmental injustice. Levels of participation in citizen science range from ‘citizens as sensors’ (crowdsourcing) and ‘citizens as interpreters’ (distributed intelligence) to levels where participants are more involved in problem definition and collection protocols (participatory science) or are even part of the entire development of the scientific process (extreme citizen science).
We must consider the necessary training and scale of facilitation roles for citizen science to combine practical and theory-based knowledge for specific types of research facilitators. This means improving facilitation by learning first-hand about specific how-to guides such as design thinking, collaborative project management in combination with existing methodologies and practices from the social sciences (such as PAR). We must involve citizens and communities in a way that enables them to design and implement, jointly with scientists or in an autonomous way, valid and robust research processes.
Accessible visualisation tools can provide a better understanding of participatory research processes and outputs in terms of scientific data, as well as open online platforms for public deliberation on the interpretation of scientific results. Data visualisation is helpful for feeding analysed data back to participants and for presenting results to policymakers. Reaching consensus regarding derived actions can also benefit policies and policymakers. Play I elaborates more on the role of policymakers.
This Play lays down action strategies to be implemented in order to make inclusive participation of communities and individuals technologically feasible. The following challenges and associated play and sub-strategies offer a set of solutions and possible best practices that emerge from existing case studies.
Diagnosis of challenges – How does the lack of technological feasibility inhibit participation in the stewardship ecosystem?
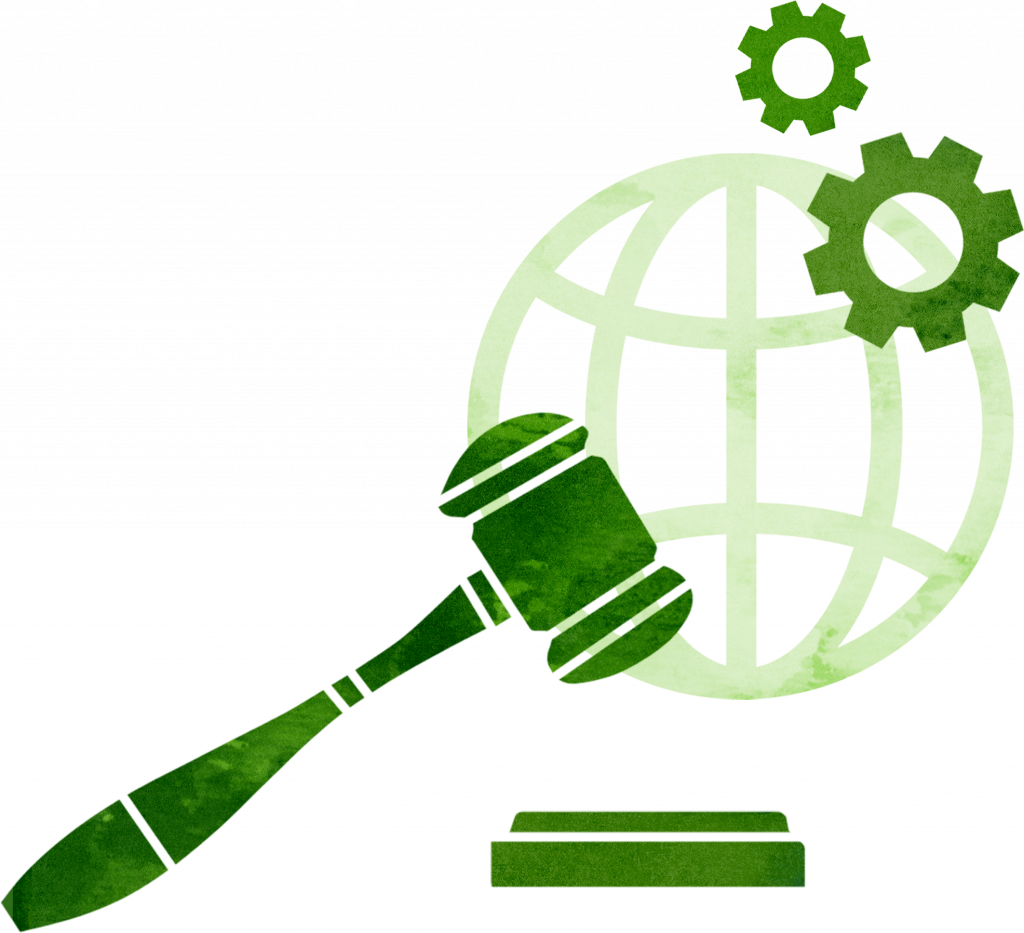
Challenge e2.1
Furthering of environmental injustice owing to technical barriers that prevent communities from being involved in the design process of citizen science projects lead
Majority of climate studies (87%) practise an extractive model in which outside researchers use Indigenous knowledge systems with minimal participation or decision-making authority from communities who hold them. The lack of information and inability to use technology due to insufficient access and proficiency in the language, cultural barriers and lack of trust can inhibit interaction and consequently lead to social exclusion.
Moreover, this can lead to lower quality data when there is a lack of adequate representation. Therefore, we need to involve citizens and communities in a way that enables them to design and implement valid and robust research processes, jointly with scientists or in an autonomous way. For instance, the easyRights project developed a Co-Design Approach Service design that introduces human-centred approaches to designing or redesigning services. This contains service design tools and templates to support users to take an active part in the redesign of such services. These templates use visual representations to facilitate co-design participants to immerse themselves in the user-centric approach of service design and learn from it.
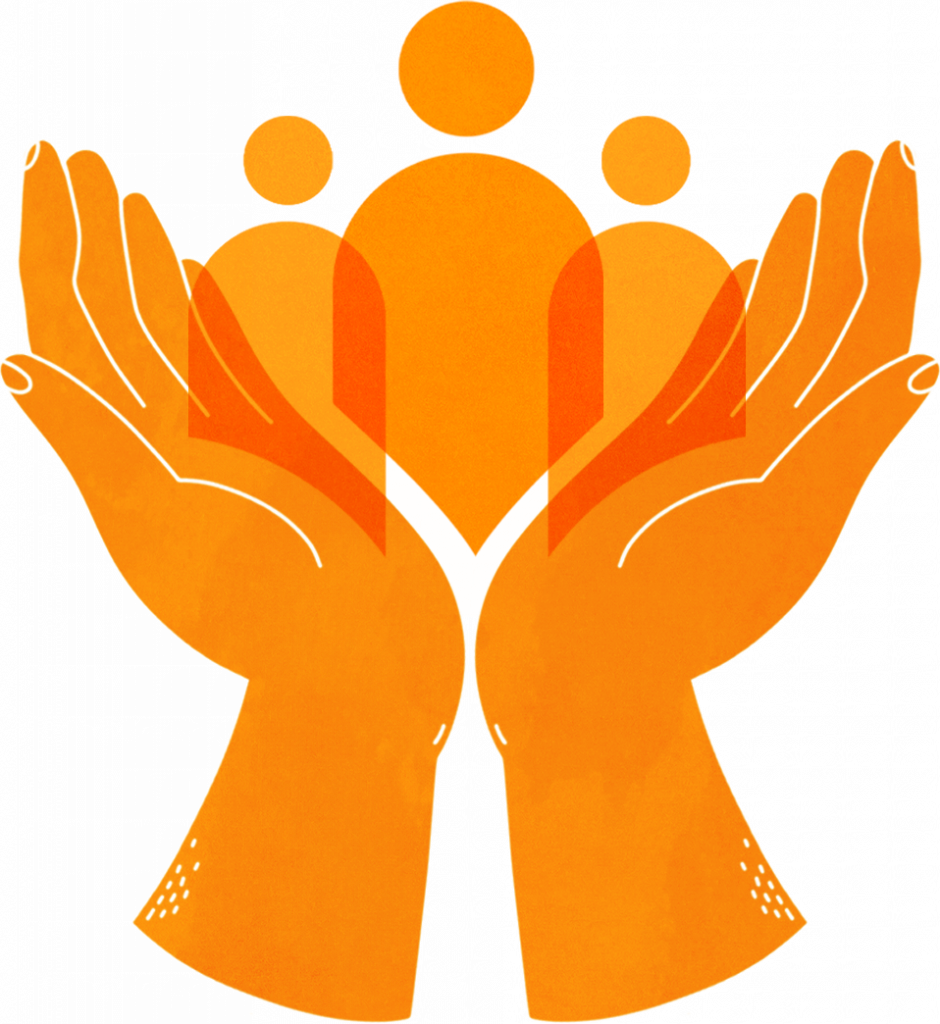
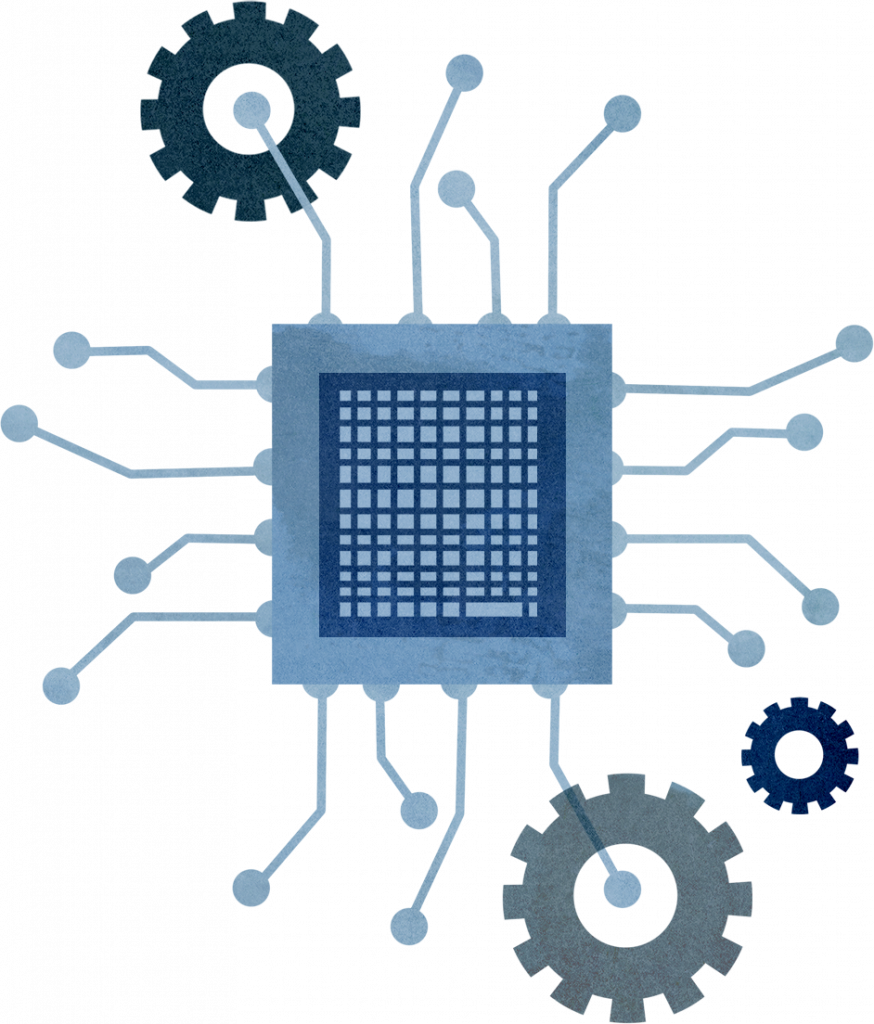
Strategy e2.1.1
Co-designing the project for full and effective participation of communities
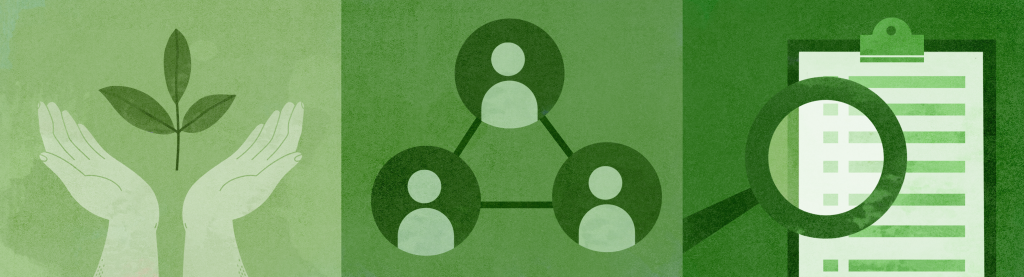
Co-designing becomes a prerequisite to include Indigenous knowledge for ‘full and effective participation of community members‘ – from design – which includes proposal development, defining research questions and objectives, implementation – which includes fieldwork and data collection to its analysis. Collaborative road mapping sessions with software companies can be conducted to ensure that all members are aligned with the code written, preventing time and resources in modifying the software to suit all needs. Further building interventions that rely on open-service architecture, enables the community members to easily take over the role of designers. Co-designing can also be done in a way where the citizen is prioritised by reactive scripting for design by placing them as the ‘designer in the loop’ thus, relying on their ability to exchange information and knowledge.
Example
One way to engage communities in a way that there is collective decision making is by co-defining problems at the outset through generation, review, and discussion of specific research questions amongst all participants. For instance, STEM4youth uses a participatory design to enable co-defining. Further conducting iterative validation mechanisms for the results of each phase such as voting on proposals. These iterative mechanisms help encapsulate community desires and local knowledge which could be recorded to assist decision-makers in applying these community preferences.
Open systems developed tools for knowledge generation, each associated with a reflexive research stage. The tools were tested and refined during six co-creative processes using a series of activities based on a research design principle – alternate phases of divergence and convergence.
This method allows ideas to be generated in a participatory way in the first sequence and in the second sequence, the participants jointly select options; through pooling and decision-making mechanisms such as dotvoting, or dotmocracy, and thermometers of concepts.
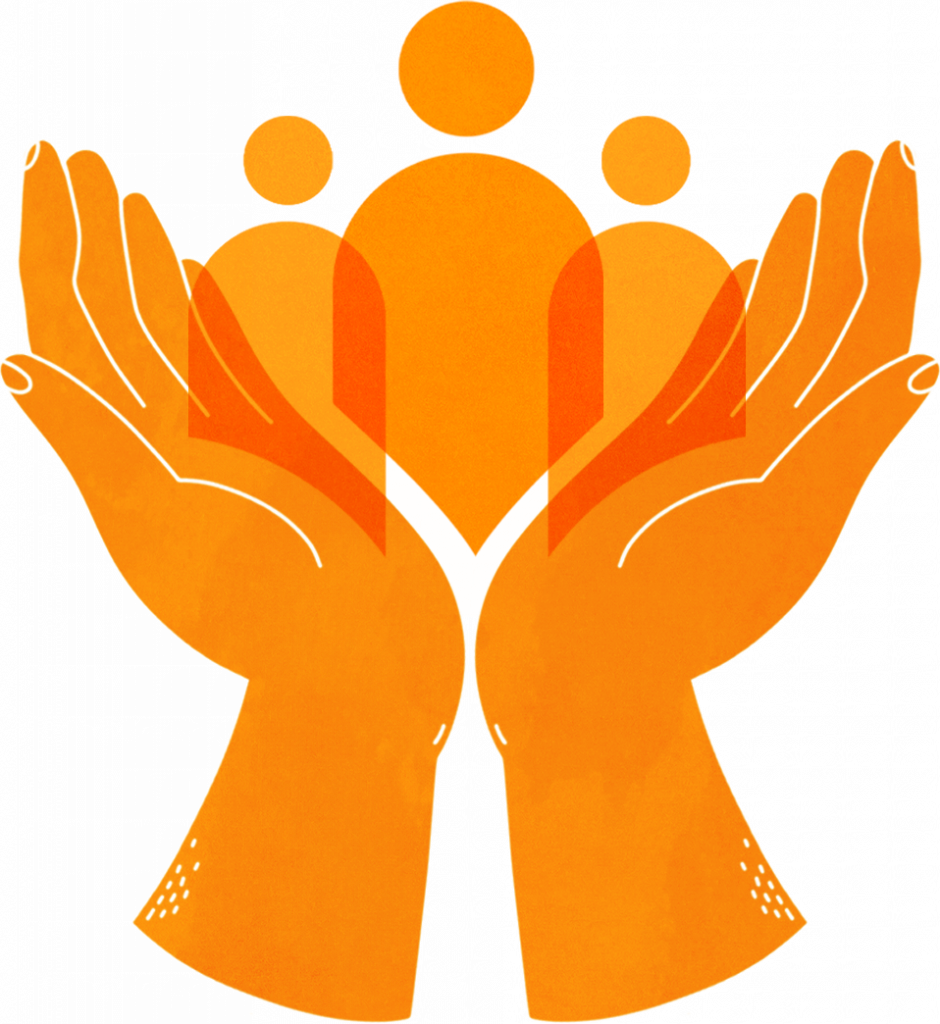
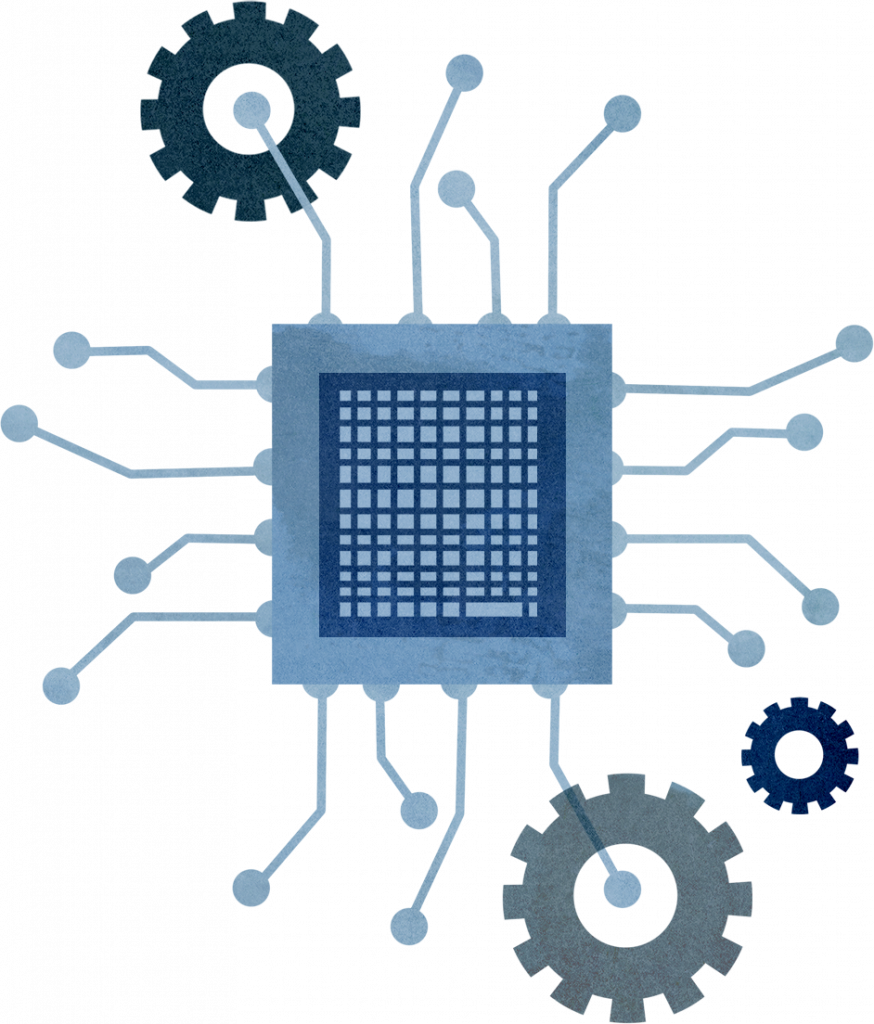
Strategy e2.1.2
Incorporating indicators/tools that ensure responsible inclusion of communities
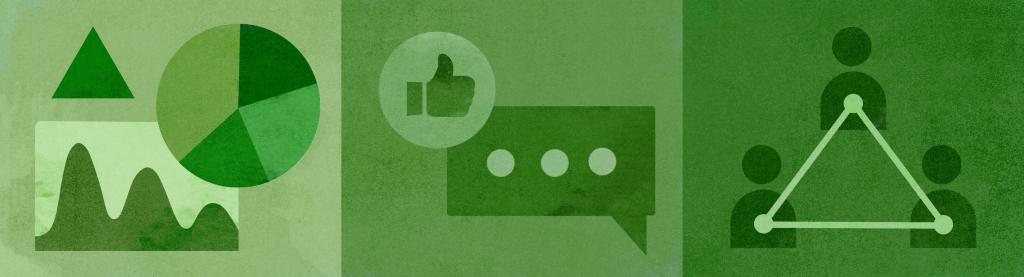
Diagrams, canvases, and gamification techniques can be used to channel citizens’ social concerns and needs into the research process. These facilitation mechanisms, such as user feedback for different types of volunteers in co-created research designs provide opportunities for people lacking a voice to reveal otherwise hidden or contentious societal problems. Providing online forums to develop research topics and define priorities along with conducting on-site community workshops could prove useful to local communities, policymakers and citizen science practitioners alike.
Example
Open Humans is a community-based platform that enables personal data collections across data streams, giving individuals more personal data access and control of sharing authorizations, and enabling academic research as well as patient-led projects. Open humans has 3 themes across its authorisation flow – members, projects and data objects. A Member can join Projects and approve them to read/write/grant access to their data. These Projects can be created by any member and a community review process was developed for Project approvals going forward; new projects are shared with the larger community for public comments, inviting feedback from all members.
Members of Open Humans can also participate in the approval of new projects that want to be shared on the platform via a community review process.
The Balloon Mapping project by Public Lab provides low-cost technology for communities to create high-resolution landscape imagery with various applications, such as to evaluate the effectiveness of bioswales in absorbing pollutants. The Balloon Mapping tool served as the technology infrastructure that supports community activism without the need for extensive ongoing expert assistance following its deployment.
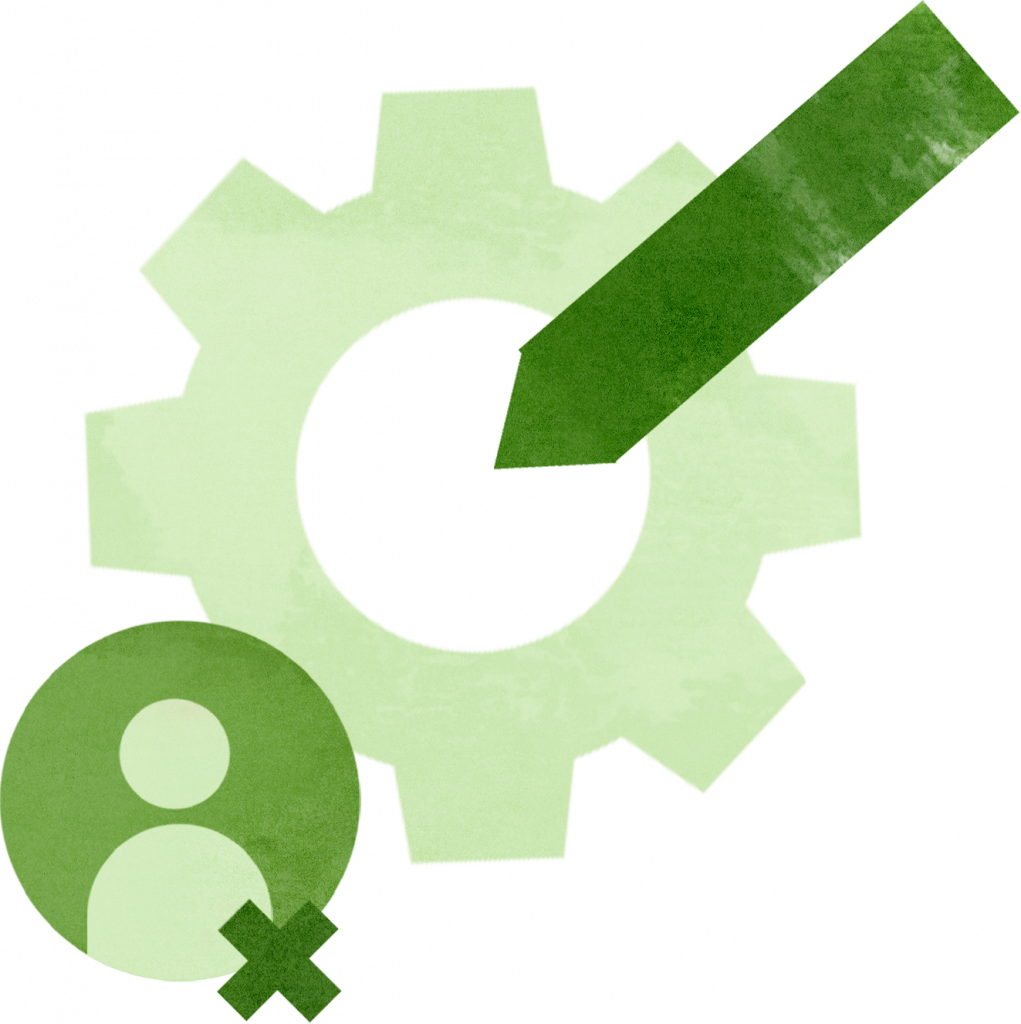
Challenge e2.2
Implicit design bias can create digital divides and barriers to access technology
The chosen technology and its design can inhibit participation based on the skills needed to use it. The more skills necessary to participate, the less likely it is that people are to be able to participate in a meaningful way. On the other hand, choosing a process that relies on sustained and intensive community engagement inhibits people from joining later on, as these people have missed part of the process. Therefore, this urgently calls for adoption of tools and practices that support the most valuable part of any system — the people within it.
New technologies need to be adaptive to lead community actions in a successful manner – such pathways include the creation of citizen science apps, the development of guided field trips, gamification, role-playing, virtual fairs, data visualisations, or demonstrating processes that are otherwise hard to observe. For instance, VR (Virtual reality) enables critical learning opportunities in an entertaining and engaging process. A study discovered that an immersive and accessible VR environment allows learners to collaborate and provides exciting opportunities in science education and public outreach (EPO) practices.
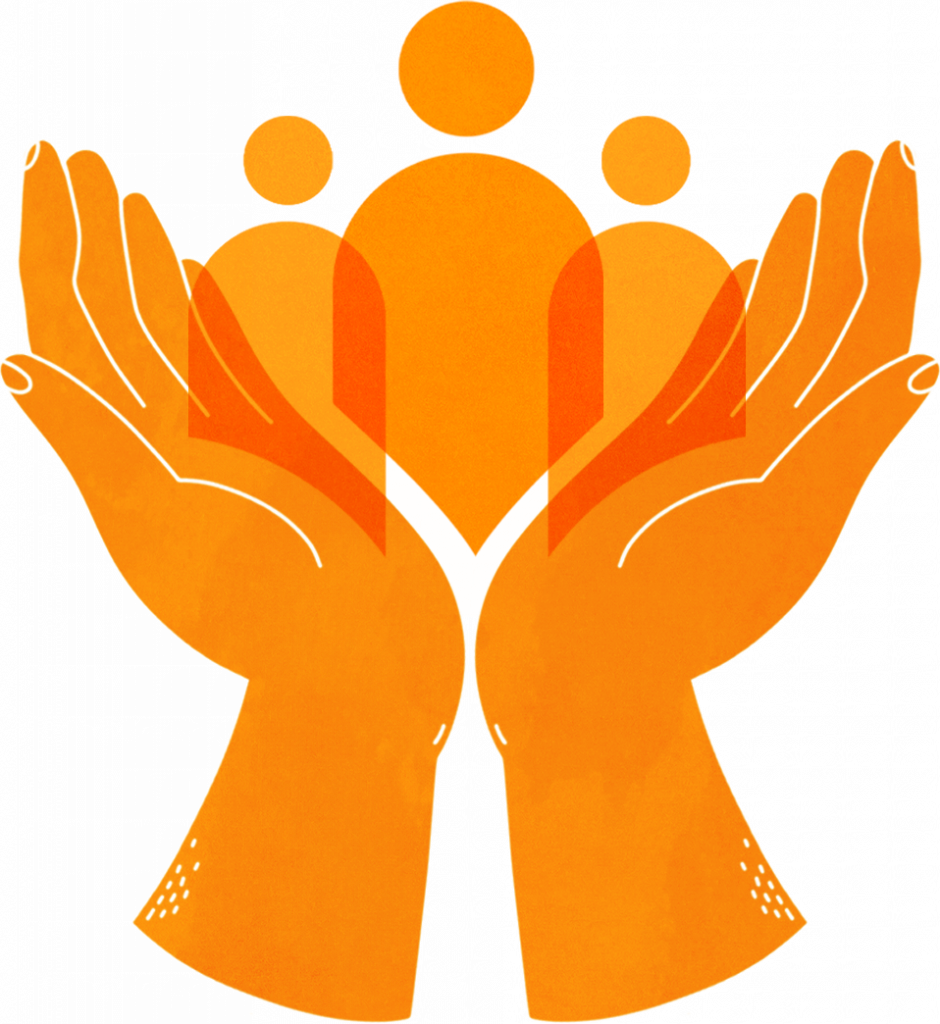
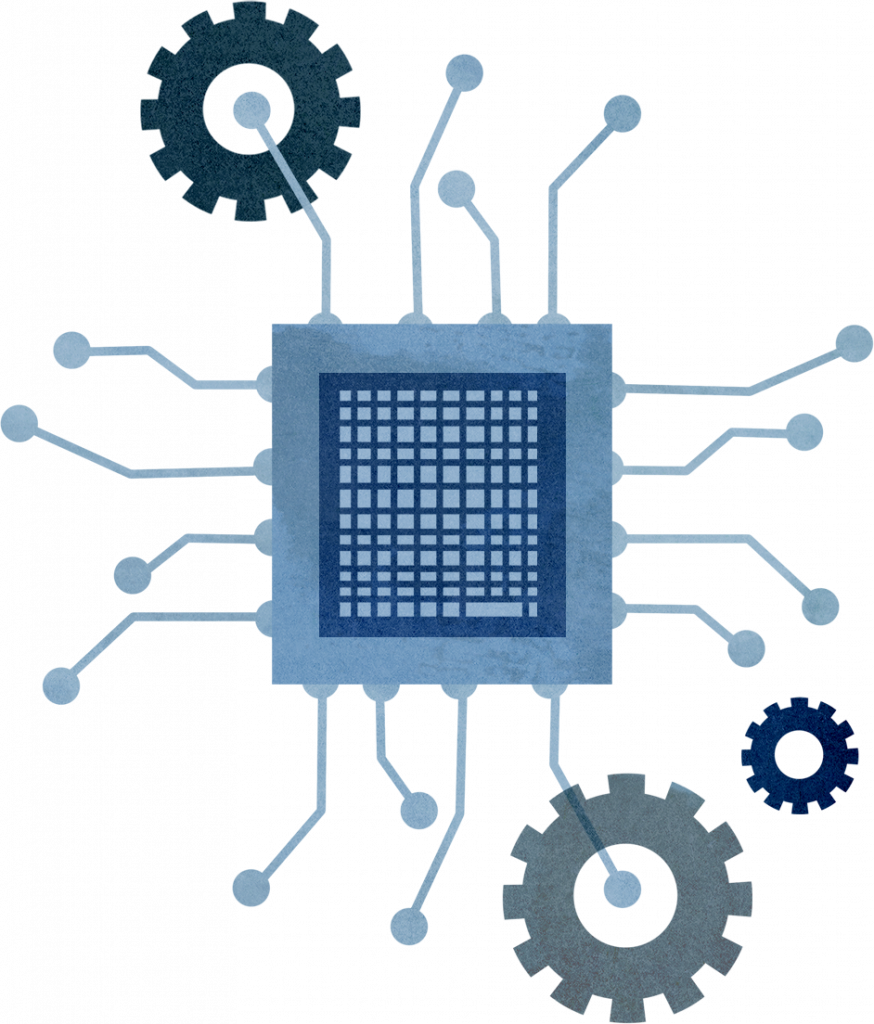
Strategy e2.2.1
Incorporating best practices and tools that enable responsible inclusion of communities
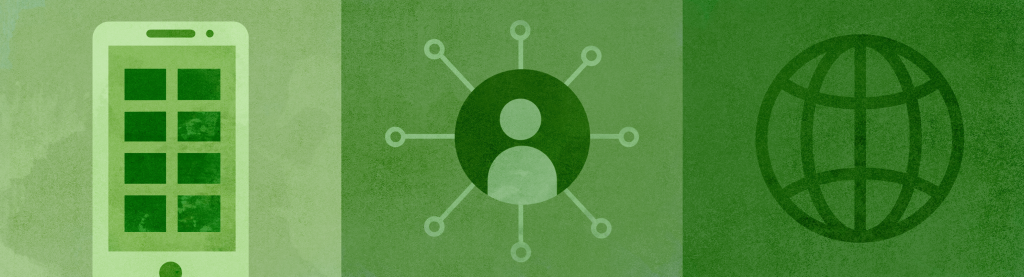
Citizen science projects must ensure visibility such that data collected by a particular individual should at the very least remain visible to that individual. For instance, TestMyBrain provides visual approaches for data analysis – by allowing participants to sift through images to perform tasks such as matching/classifying images. Virtual citizen science projects like EyeWire uses real time communication systems or forums to provide better support to the community.
For citizen science initiatives to function, greater usability of apps and the support provided (such as personal training) to participants are influential factors. The data infrastructure needs to be flexible enough to accommodate the diversity of data types such as text/tabular/geo-spatial and various media types – iNaturalist is a good example of one such biodiversity aggregating platform. Platforms such as iNaturalist and Natusfera allow citizen science volunteers to find biodiversity monitoring projects and directly upload biodiversity data. Citsci.org allows participants to create their own citizen science projects to initiate data collection and analysis via websites and/ or mobile applications. Other platforms such as Zooniverse, provide cyberinfrastructure supporting data analysis via tasks such as classification, annotation and tagging.
Example
Web Accessibility Initiative provides web accessible tools training that web developers should aspire to meet. Tools that contribute to the design and development of components of FAIR infrastructure and platforms can be used for responsible data management planning. The conceptual model from the COST Action CA15212 was established to improve data standardisation and interoperability in citizen science activities. It utilises past models and contributes to current standardisation efforts, such as the Public Participation in Scientific Research (PPSR) Common Conceptual Model and the Open Geospatial Consortium (OGC) standards. Open Humans encourages project administrators to be clear about both data management and security in a thorough community guide including best practice details on how to communicate to participants which data access is being requested and why in clear and plain language.
The Project BudBurst website, for example, trains participants to collect and publish data and provide education materials. The project also supports a mobile application mainly designed to facilitate data collection. Citizen developers allows for people with little or no coding experience to write their own applications through the use of low code or no-code technology. The increasing availability of development frameworks help project owners create websites and other tools to support citizen science projects without the need to write complex software from scratch. At a fundamental level, WordPress, Django, Wix and Weebly are examples of frameworks that provide means for interacting with participants and also support responsive design to deliver content appropriate for display on mobiles, desktops and tablets. For more advanced users, frameworks such as PhoneGap and Ionic help developers write websites in HTML and JavaScript. Responsive design enables websites to be viewed according to the device being used to access them, by adapting layouts, media items and other content to different resolutions and screen-sizes. For projects that seek to host a website and a mobile site or app, styling tools such as Bootstrap and Boilerplate help simplify this process.
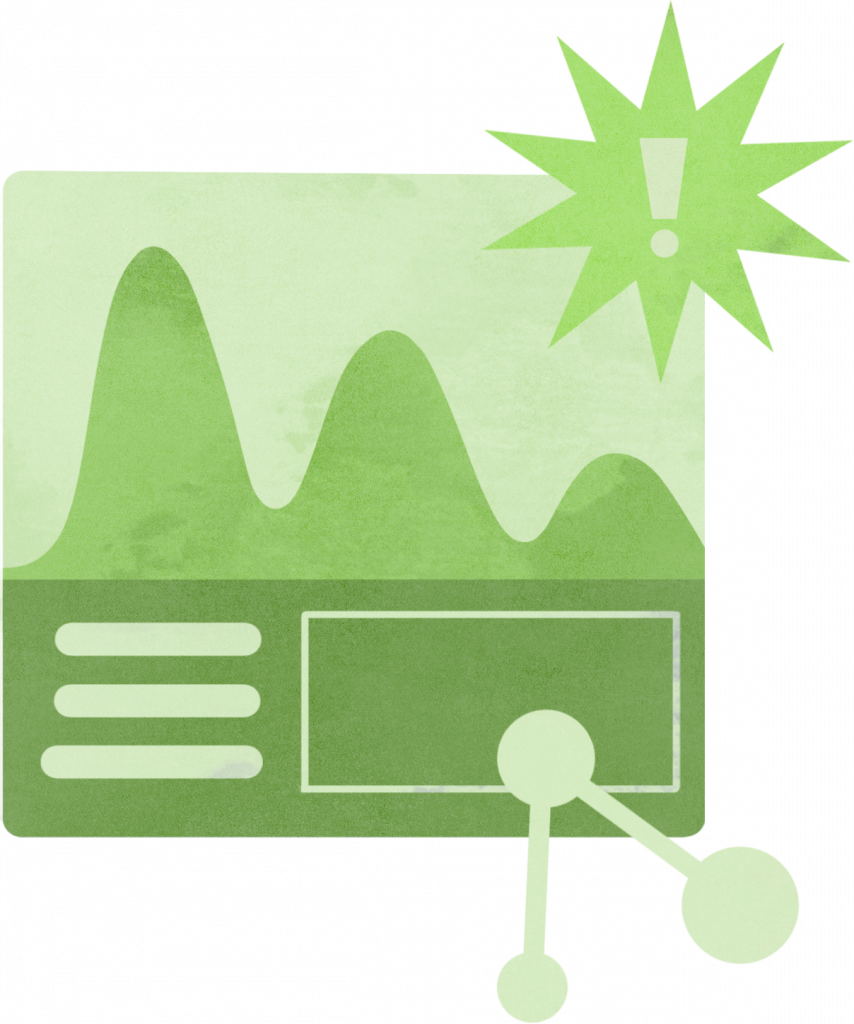
Challenge e2.3
Lack of quality data and validation mechanisms can result in its misuse and distrust that would render such data unsuitable for research or policymaking
Data from citizen science is immensely valuable as it provides evidence that professional science cannot gather. However, aspersions cast on the collection method and its validity by citizens is the main reason that it faces distrust and scepticism from scientists and policymakers. The misuse of data is likely to occur when there is inaccurate data documentation, this could lead to overcorrection which can cause more errors and ultimately exacerbating distrust and suspicion of all citizen science data. This has caused many to discard it as unsuitable for research purposes. From a more general research design perspective, the validity and the reliability of data are most important. Datasets should be consistent and must have adequate distribution of the target population area. Especially in citizen science projects operating large datasets; reliability and quality ensure trust and aligns with policy requirements and stakeholders’ interests.
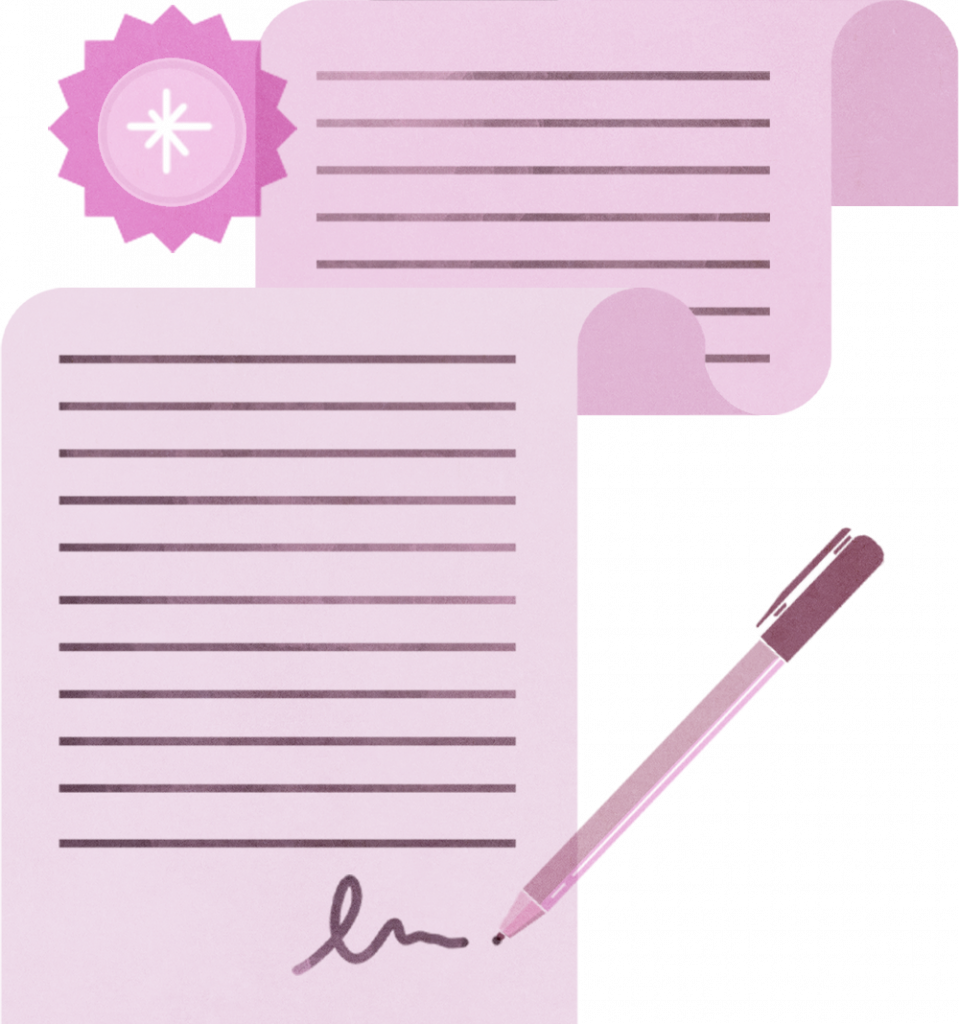
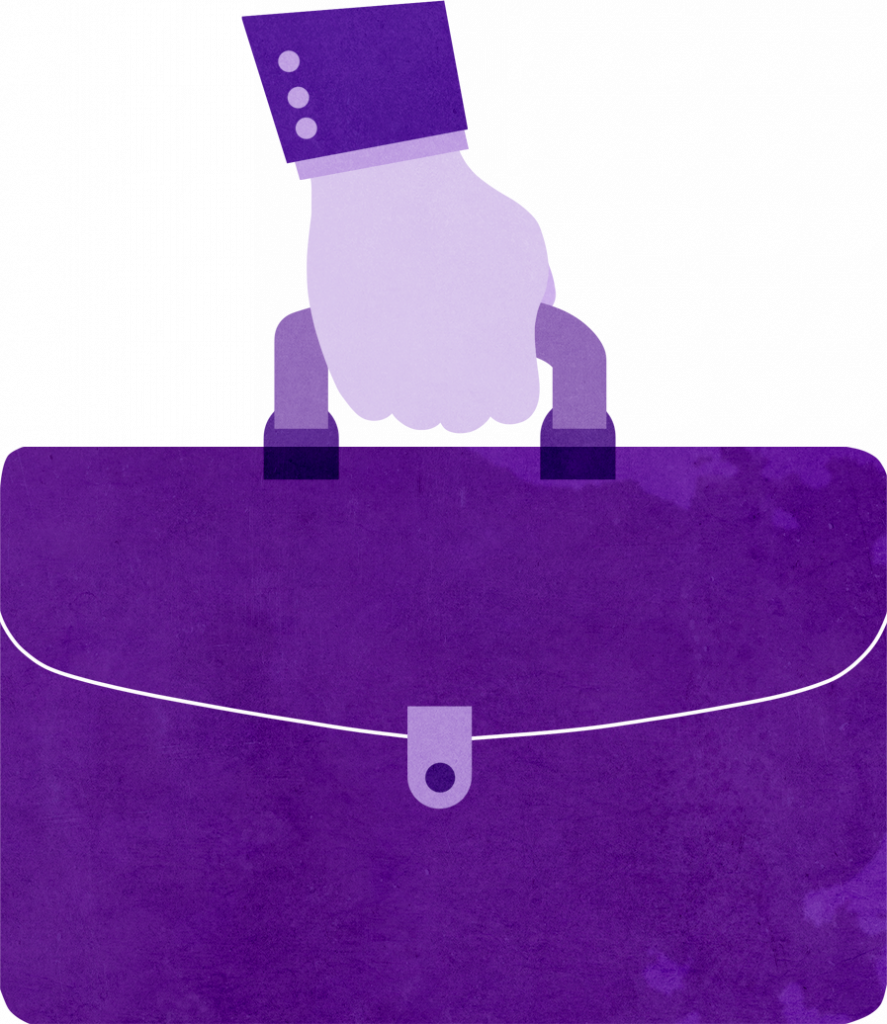
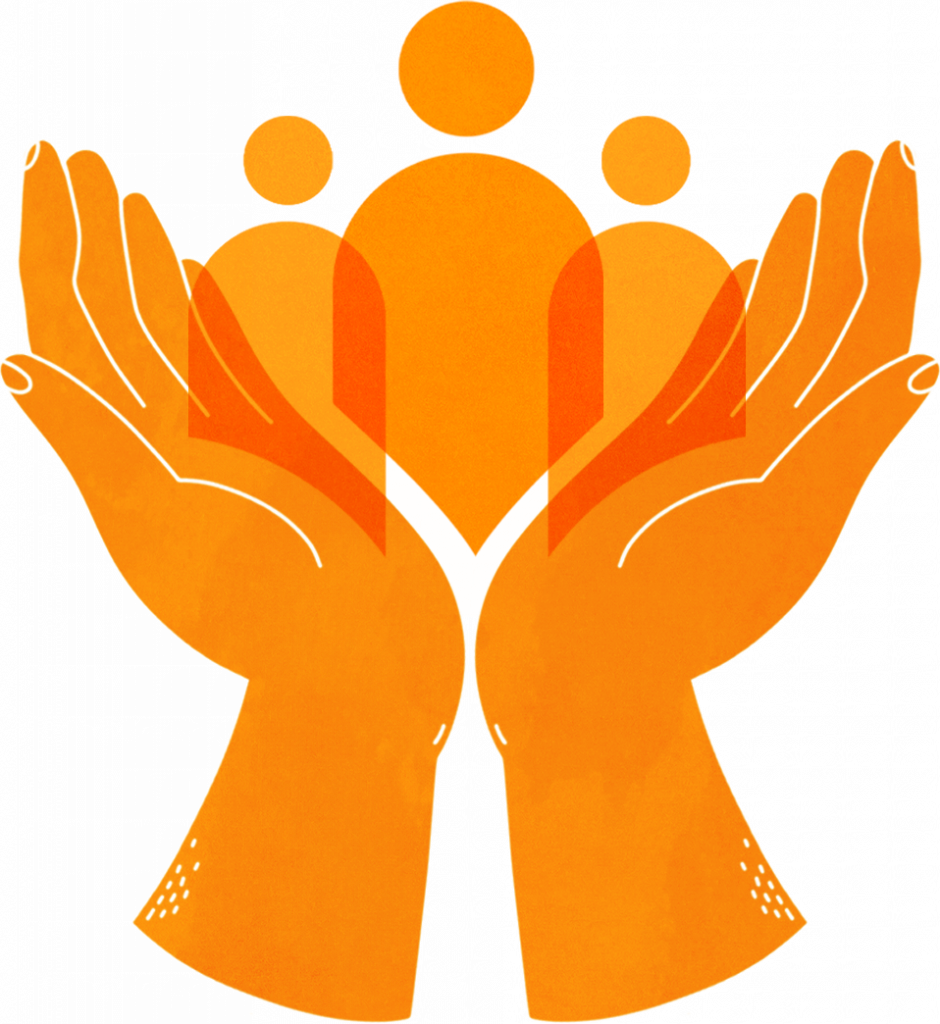
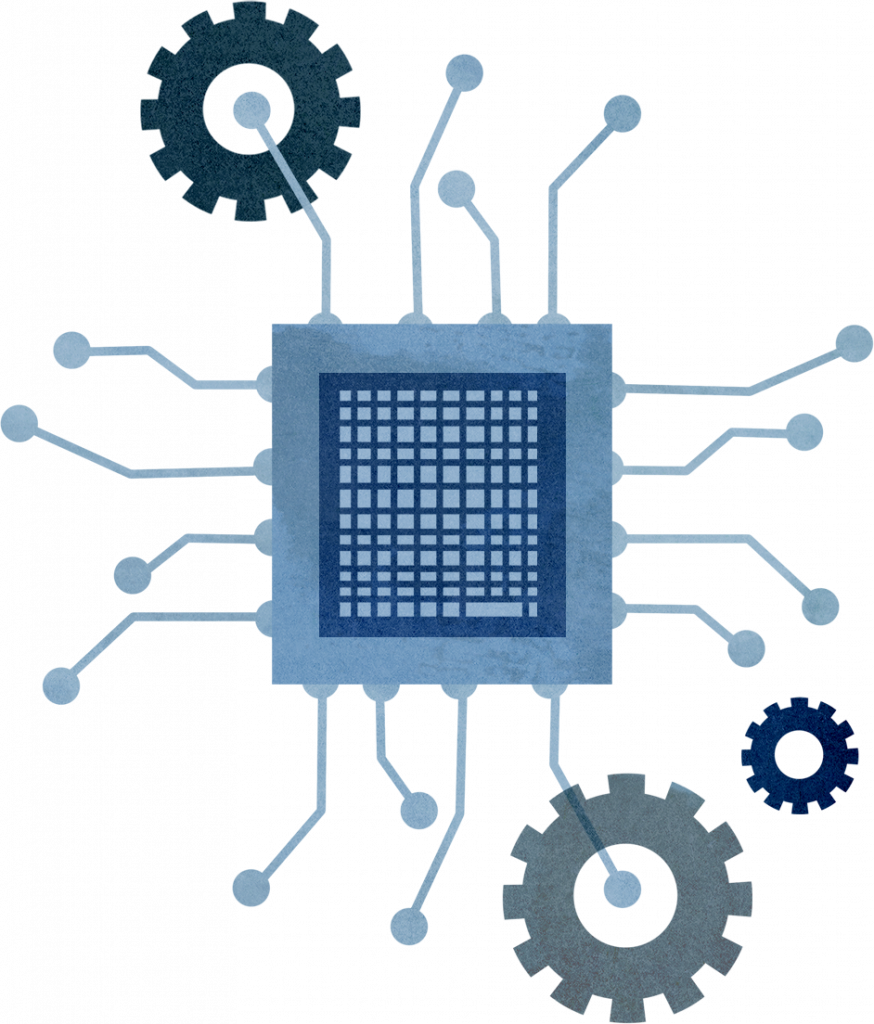
Strategy e2.3.1
Maintaining data quality, employing verification mechanisms and contextualising data
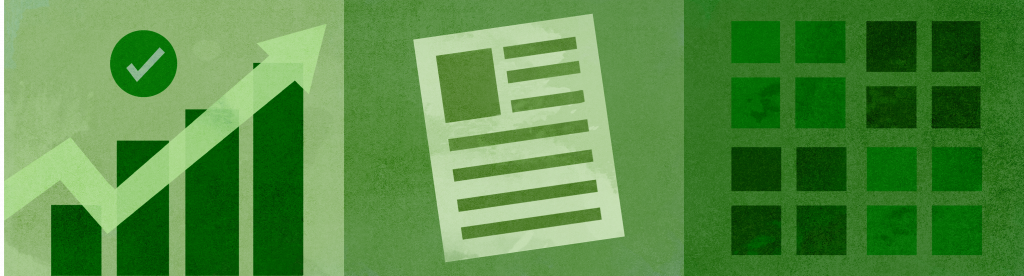
To ensure a minimum standard of data quality, a plan or protocol of data collection must be set out at the start of a project. This is to manage different levels of expectations of data quality from different stakeholders. All stakeholders should be invited to co-develop standards for data quality and explicitly state the data quality levels they expect in order to form an agreed approach to data quality (citizens, scientists, researchers, funders and policymakers) through online forums.
Contextualisation i.e., communicating the context in which a particular high-volume data set has been created enables data re-use. Metadata, attribution, and curation are the most prominent examples of data contextualisation. Appropriate documentation and metadata are the most effective deterrents against using unsuitable data. Metadata helps create contextual information – can include title, number of participants, contact details, data lineage and geographic extent of data. This helps in assessing the quality of data as data points such as identity of observer or location accuracy can be determined. Further, contextualisation and creation of metadata becomes extremely relevant when this dataset is applied for another purpose. When these datasets are combined with others to be re-used for a different purpose (like policymaking), the context needs to be carefully examined.
Preemptively restricting data inputs can help identify unforeseen sources of errors or other problems that can be fixed before the project starts. This can be done through methods such as profiling which helps the data collectors to understand the quality challenges and pre-testing by gathering sample data before a citizen science project begins using both expert and beginner contributors. When new data is submitted, effective data validation mechanisms need to be implemented such as averaging data records, flagging errors and providing feedback to the users. These mechanisms can improve the quality of data and user trust in the data.
Automatic quality assessment involves the use of software based systems to carry out a quality assessment of the data generated or collected by a citizen science project. There is a wide range of approaches, such as data mining algorithms, which filter and search for problematic data, statistical analysis (plausibility of data), and qualifying systems.
Model-based quality assessment goes beyond automatic filtering technique and tackles residual errors using an explicit model of how the phenomenon of interest is expected to vary in space or time. Examples include de-biasing procedures and generation of contributor ratings, based on identified sources of systematic errors in the archive of observations.
Example
The PDI laboratory information management system (LIMS) tracks samples, receives instrument output, stores metadata, and produces chain-of-custody certification, thus standardising and automating processes. For instance, in Puerto Rico a data collection app (for animal identification) was developed by PDI (Partnerships for Data Innovation, which provides scientists with state-of-the-art tools for robust data management) wherein data uploaded could be accessed instantaneously by scientists and was extremely effective in automatically calculating proper dosage and other relevant information – reducing post processing time and the margin of error.
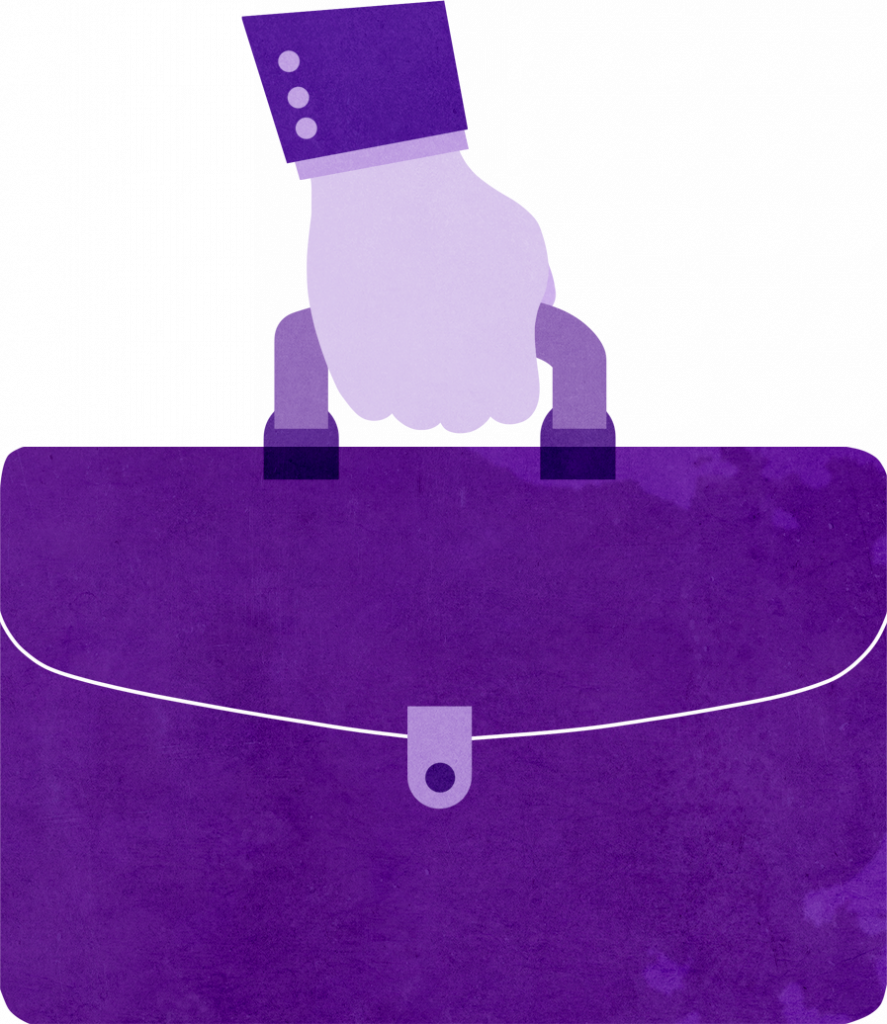
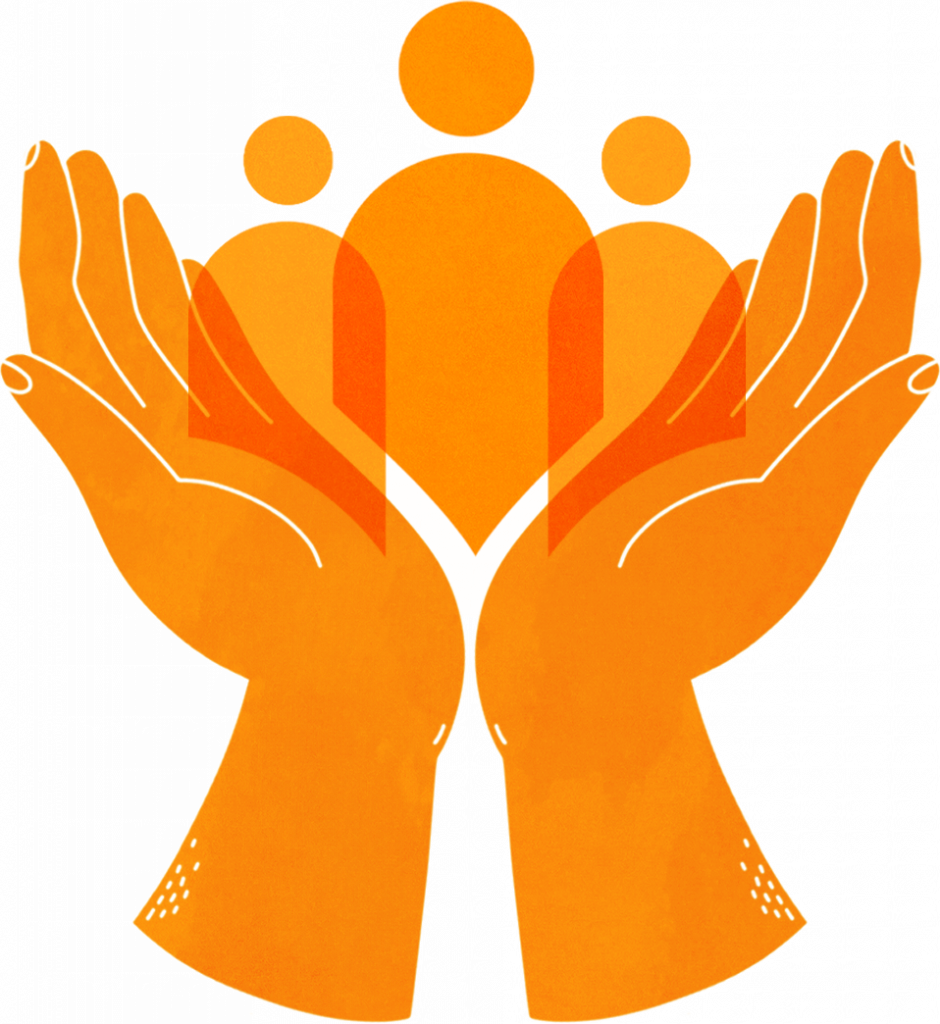
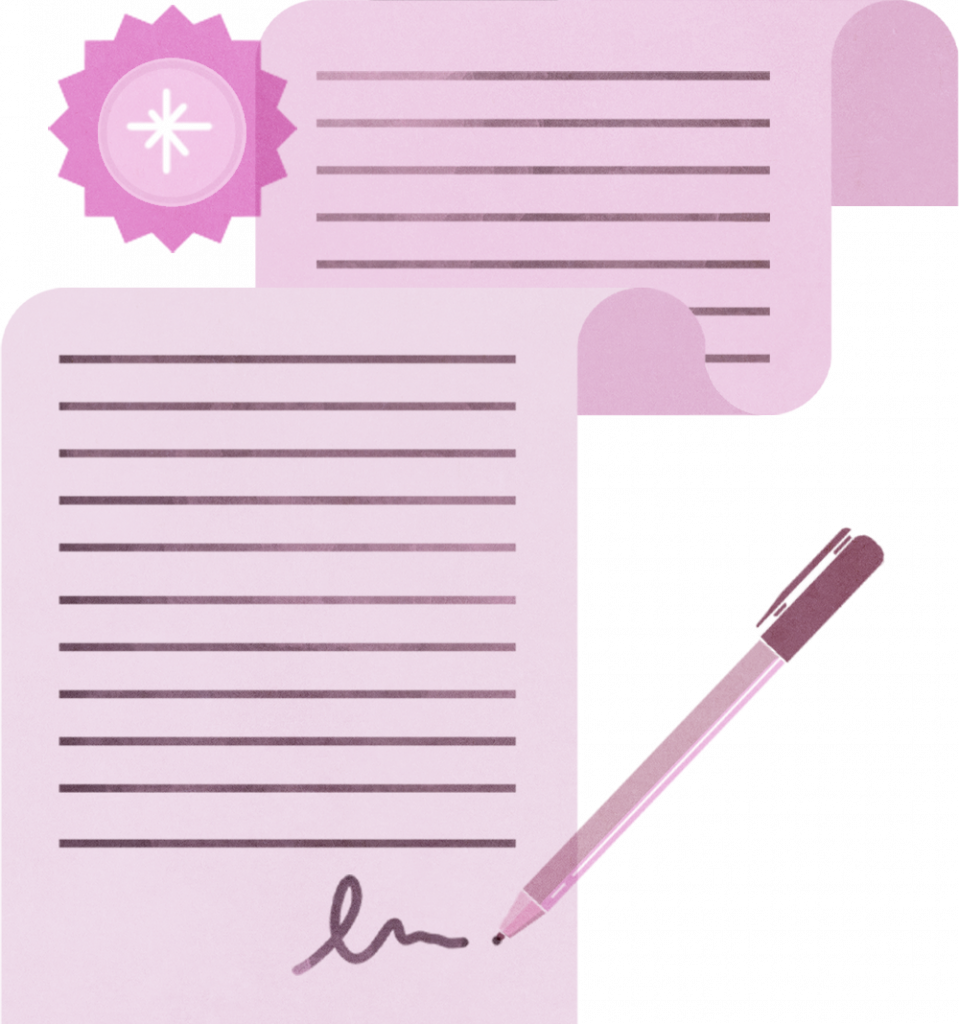
Strategy e2.3.2
Involving participants in data collection methods
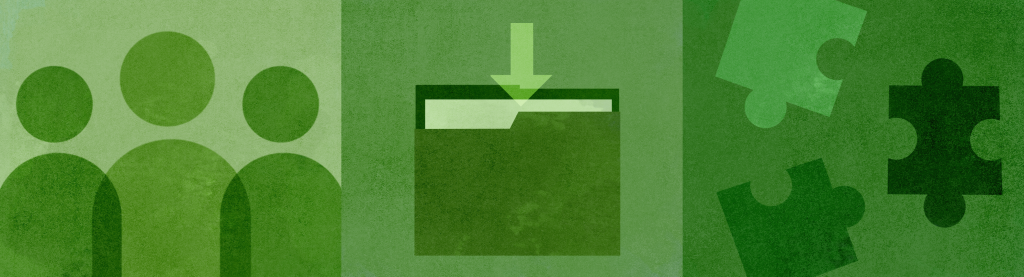
Comprehensive data collection protocol that covers all stakeholder expectations must be implemented. Poorly designed or overly complex protocols can also create skill inequality if some protocols assume a specific level of scientific training before they can be used carrying the risk of excluding whole communities. A possible solution is to introduce a permanent channel or forum that participants can use to contact creators and provide input. Tasks of data collectors can be made more straightforward by pre-filling files with often used values and providing examples for how observations are recorded. These data collection tools need to have a simple user interface design and must be compatible with a variety of skills and objectives of potential citizen scientists. Integrating simple and affordable visual ways by use of images and gamification features to communicate concepts which are often complex or specific to the scientific world.
Programs with very simple data collection methods report much higher rates of participation, but data is often biased and noisy. Therefore, using simple techniques such as data collection forms or semi-structured surveys reduces the need for rigorous training, at the same time ensuring that data is collected in a prescribed format. For instance, Ushahidi and Open Data Kit (ODK) provide a way to easily develop customised surveys and set up websites and mobile applications that can be distributed to crowdsource information. These frameworks also allow project owners to aggregate, visualise and analyse the data collected.
There are a range of tools available to support project coordinators and volunteers in processing, analysing and visualising data – Earthwatch’s Freshwater Links and UCL’s Extreme Citizen Science: Analysis and Visualisation (ECSAnVis) are examples where users can visualise data coming from a variety of remote databases. The ECSAnVis project focuses on the creation of software called Sapelli that runs on mobile phones and allows non-literate people to collect information about their area with an app that participated in the design that is suitable for such users. This software was tested on the community and was built considering those who have little or no understanding of tech, but could still fully take part in the process of building research questions and collecting data.
Example
The main tool for data collection in D-NOSES to collaboratively build odour maps is the citizen science open app OdourCollect. Communities affected by odour issues can map them collaboratively so they can be viewed by all. The project enhances digital inclusiveness by creating tools for ensuring inclusiveness in specific social environments : for example, for the elderly or people with difficulties in accessing digital technologies. The D-NOSES methodology seeks to empower citizens and key stakeholders to generate, access, and use data related to odour pollution. The collected data is then used to inform and co-design possible solutions to manage odour problems
Data collection tools by SPOTTERON provides for data infrastructure including handling and storage – data analysis toolkit